Interview • Predicting the truth from hybrid imaging
Holomics: a trendy but complex topic
‘Is it possible to know whether a treatment will work before even starting it – in other words, to predict the truth? That’s the great promise of holomics, a concept that everyone has been involved in without even noticing,’ said leading French physicist Irène Buvat, from the In Vivo Molecular Imaging French lab, who is set to focus on this subject at ECR 2019.
Interview: Mélisande Rouger
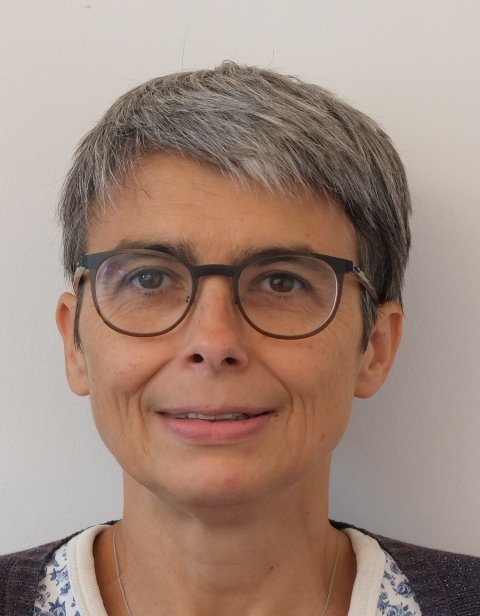
‘The truth,’ said physicist Irène Buvat, discussing whether a therapy prediction could work, ‘can involve several things: the patient’s response to therapy, the prognosis, patient tumour molecular subtype, dementia type, the presence of a genetic mutation – usually something that’s clinically or biologically relevant. We call it truth because we want to make a prediction that will prove to be 100% accurate.’ To predict the truth, one has to account for a number of variables, such as abnormalities seen in blood samples, genetic mutations, the patient’s age, condition, medical history, etc. And of course, imaging data, which are very useful and currently absolutely needed for patient management. This is especially true for hybrid imaging data, Buvat pointed out.
What are holomics?
‘Holomics can be defined as the gathering of genomic, radiomic, proteomic, clinical, immunohistochemical and many more data, and their integration in predictive or prognostic models. Holomics can be seen as an extension of radiomics, which is the extraction of features from many images as a foundation for predictive models. In short, holomics is radiomics enriched by other types of data. In contrast to current predictive models, holomics includes all these different types of information to predict the likelihood of a patient responding to therapy, developing a certain disease, and so on. The assumption is that information reflected by images will tell us more if they are put into context, i.e. if interpreted together with other non-imaging data.’
Recommended article
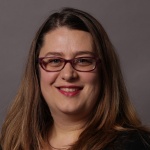
Article • Post-hypothesis analysis
The mechanics of radiomics
Confirming or infirming hypotheses has long driven scientific research; however, this traditional and costly approach is giving way to data-driven initiatives, according to Prof. Laure Fournier, a leading radiologist at Georges Pompidou European Hospital in Paris. “Usually we formulate the hypothesis first, then take an image and analyze it. We like that in France, it comes from Descartes. The…
What are the potential benefits?
‘Being able to predict will definitely contribute to precision medicine, because if we can predict that a patient has a very low likelihood to respond to a given chemotherapy and there is an alternative treatment, that treatment will be given first. Patient management also heavily depends on the experience of the specialists involved. The idea with holomics is to erase this difference by integrating different kinds of information on a quantitative and reproducible basis to assist therapeutic decisions. Any decision would of course be made under the control of a specialist, but the algorithm would always provide expert knowledge.‘
Where is it interesting to use holomics?
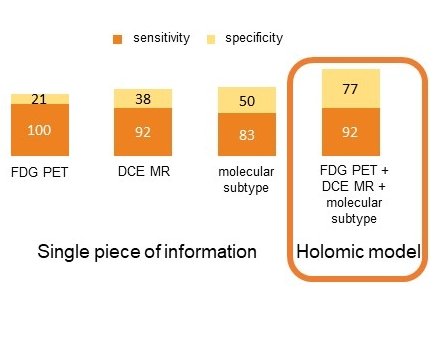
‘Holomic approaches are mainly being developed in oncological imaging, but are also valuable in cardiovascular and brain imaging. Right now, people creating scores involving image-derived parameters and other patient features like age are doing holomics without noticing. The word was first coined in the context of tumour imaging. Holomics is a trendy, but complex topic. We have a few results combining blood markers with imaging markers, but we could have far better results if we could add information from genomics and other fields.'
Why is it appealing to implement holomics in hybrid imaging?
‘The more different the imaging data, the deeper we can use holomics. Hybrid imaging gives information from two imaging modalities in the same machine at the same time, which makes it a privileged gateway to collect many distinct types of information. PET and MR offer complementary but non-redundant information that can give a more complete profile of the disease. We can see anatomic characteristics on MR and, at the same time, metabolic features on PET, and additional functional features using different MR sequences. The main obstacle now is to collect enough reliable and rich data to build holomic models. There are fewer hybrid imaging modalities, but the information they provide is richer than CT or MR scanners alone. In hybrid scanners, images are perfectly aligned in space and time, unlike two exams performed on two different machines at different times.’
How exactly do holomics work?
The overall current challenge is to make the most of these different approaches to integrate medical images and associated data, and produce new knowledge that will help the medical doctors to optimise patient management
Irène Buvat
‘We use both shallow and deep learning, which differ in the way information is extracted from the data. To design a model, three types of learning techniques can be employed: supervised, unsupervised or reinforcement learning; supervised and unsupervised being by far the most frequent now. As an example for supervised learning, we tell the algorithm which tumours in a data set are benign and which are malignant. Then, the machine learning approach will find the model that makes the most accurate prediction for the data present in the training set. Unsupervised learning, on the other hand, is performed to try and identify trends or structures in any given data. Often, clusters can be identified, i.e. groups of data that are similar within each group and different between groups. This approach is very useful because it might reveal trends that we are completely unaware of and might give rise to new hypotheses. In reinforcement learning, we do not provide the algorithm with examples of images associated with the right class they belong to, but we give feedback in the form of reward or penalty. This approach mimics the way humans learn. Therefore, the overall current challenge is to make the most of these different approaches to integrate medical images and associated data, and produce new knowledge that will help the medical doctors to optimise patient management.’
Profile:
When Irène Buvat gained her PhD in Particle and Nuclear Physics from Paris Sud University, France, in 1992, the physicist oriented her path towards nuclear physics for medical imaging applications. After a year at University College London, UK, and two more at the National Institutes of Health, Bethesda, USA, in 1995 she joined the French Centre National de la Recherche Scientifique. Buvat now heads the In Vivo Molecular Imaging research lab at the Service Hospitalier Frédéric Joliot PET centre in Orsay, where her research focuses on developing quantification methods to make the most of SPECT and PET data coupled with CT or MRI, to understand biological mechanisms for optimising patient management. She is involved in radiomic and holomic approaches to further enhance the role of PET/CT and PET/MR in precision medicine. Ever active in training students and knowledge and software dissemination, she promotes open-access to high-standard research material.
26.02.2019