Source: Shutterstock/LuckyStep
Article • Digital pathology
Bridging the gap between pathologist and algorithm
Digital pathology can gain huge benefits from rapid image search and the effective extraction of knowledge from large medical archives via artificial intelligence (AI).
Report: Mark Nicholls
It facilitates identification of anatomical and pathological similarities, significantly enhances the clinical workflow, and ultimately paves the way for more informed diagnosis and better patient outcome. Effective archive searching bridges the gap between computer algorithms and pathologists, adding a new dimension to clinical practice in an ever-more demanding working environment. The subject was explored in the keynote presentation ‘Learning to search in large image archives: how AI agents can serve pathologists’ delivered by Dr Hamid Tizhoosh, at the Digital Pathology and AI Congress in London last December. Tizhoosh, from the University of Waterloo in Ontario, Canada, and director of the Knowledge Inference in Medical Image Analysis (KIMIA) Lab, outlined how AI uses software algorithms to represent data, classify data, and search for similar instances, either in a supervised way (with the pathologist’s direct and indirect instructions) or in an unsupervised approach.
The human operator is the ultimate validation of AI, so that means there will be no full automation and the human will always be in the loop from the beginning – either to teach, validate, accept or reject
Hamid Tizhoosh
‘Learning is just distance minimisation between what AI is calculating and what pathologists have put forward as labelled or annotated data,’ he explained. The deep learning aspect of AI has proved most effective in this respect with the ability to classify images (such as deciding whether an image is cancerous or not, or adding grading); segment by focusing on a specific part of the image; or search the archive to find similar cases. ‘Image search is the unsupervised part,’ he said, ‘because you do not need a pathologist to teach the software about what to do.’
Whilst he highlighted AI shortcomings, he also posed the question as to whether AI can ultimately replace the pathologist. ‘The answer to that is no,’ Tizhoosh confirmed. ‘AI is not at the stage where it can write a pathology report but you can use AI to search and find similar cases so that the pathologist can write a better report. The human operator is the ultimate validation of AI, so that means there will be no full automation and the human will always be in the loop from the beginning – either to teach, validate, accept or reject. ‘You cannot replace the pathologist because AI is still very weak and it is the pathologist who writes the detailed report, whereas AI just gives a simple answer. Algorithms cannot provide explanations such as why it came to a decision, or why do you think the image depicts cancerous regions. AI application is just to assist. So, asking if AI can replace the pathologist is not a serious question.’
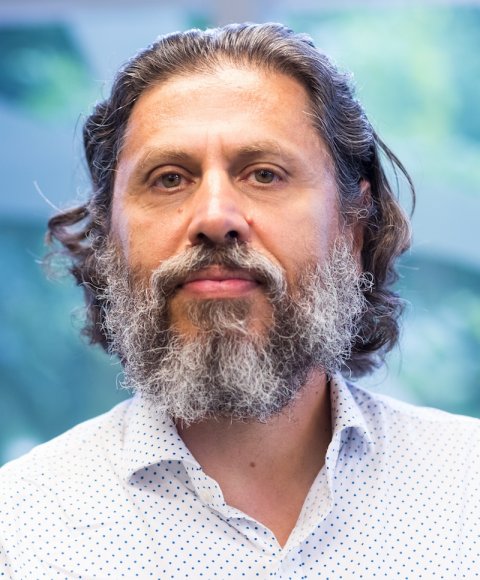
To truly have AI that can perform at this level will require it to be capable of multi-tasking and to have human level intelligence such as ethical cognition, he pointed out. However, the existing benefits are significant with AI able to rapidly search out similar cases with annotated information. A big advantage of this is the element of ‘virtual peer review’ because many of the reports in the archive will be from different pathologists. ‘AI can access the knowledge of colleagues even when they are not there, but you need a large archive of images for that, let’s say, upwards of half a million,’ Tizhoosh said. For an image search in such large archives to be effective, bar-coding (binary tagging) is the key step. ‘You can search half a million scans a second,’ he continued. ‘What you can’t do is bring up 500 glass slides from the basement and search through them – this is where we really start to think about the advantages of digital pathology and if you have a large enough archive the search can really contribute to consensus.’
Profile:
Hamid Tizhoosh is director of the Knowledge Inference in Medical Image Analysis (KIMIA) Lab in the Engineering Faculty at the University of Waterloo. He is also a member of the Waterloo AI Institute, and a faculty affiliate to the Vector Institute. As part of his commercial activities, he is presently the AI advisor of Huron Digital Pathology, St Jacobs, Canada.
25.04.2019