Article • Teamplay
Big data and computer-assisted diagnoses
One thing is certain in big data discussions: Intelligent machines will change our world considerably. What is less certain is exactly how these changes will look. Although networked data processing offers many opportunities, its development is still in the early stages.
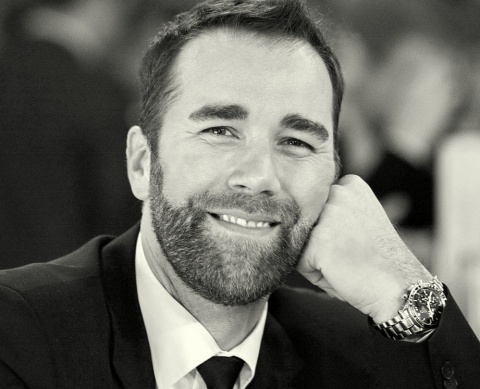
In medicine, there is great hope that it will be possible to extract and use valuable information hidden in the masses of digital data in a meaningful way. Those currently involved in the research and development of innovative technologies are likely to be among the big winners resulting from this boom. Here, Peter Aulbach, Marketing Manager at Siemens Healthcare, gives us some insights into his company’s present and future strategies around big data.
There has been some significant success in computer-assisted detection. CAD systems, such as those used for mammography and lung screening, look for typical patterns and recognise irregularities that might indicate pathological changes. ‘The software marks the conspicuous parts of the data sets at which the user should take a closer look. The objective is not to replace radiologists but to support them in their work, so that they do not overlook anything. Doctors will continue to make the diagnoses,’ Aulbach points out.
Things should become even more exciting once these artificial intelligence learning software programmes can make prognostic statements. Siemens is currently testing software that can differentiate between threatening and non-threatening coronary stenosis. The analysis programme determines the virtual blood flow reserve (CT-FFR) in the coronary vessels during a cardiac CT scan and decides whether a relevant stenosis is present, or not. In the future this could avoid unnecessary cardiac catheterisations.
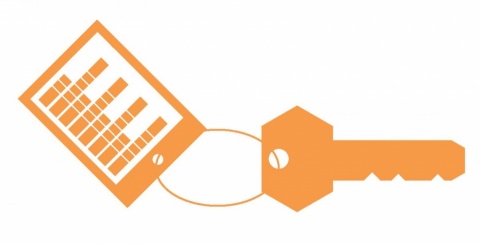
What is hardly ever mentioned in the context of the algorithms on which these learning programmes are based, says Aulbach, is image quality: ‘Achieving significant results for the analysis and processing of data requires perfect raw data. Image acquisition therefore calls for the utmost care and precision, as there may be artefacts that later cannot be eliminated. The robustness of statistical evaluation methods therefore depends on the source image.’
The marketing manager does not see a problem in contrast media administration, which concentrates in a different way in each individual patient and consequently produces different image information: ‘Dual-energy-CT facilitates mono-energetic CT imaging, which automatically balances the different intensities of contrast media concentration in different image data sets. For the purposes of comparative analysis, it’s therefore not relevant whether some data sets have higher contrast media concentrations than others.’
The big data programmes currently in use are only aimed at assisting doctors. However, at some point, and with the help of learning computer systems, there is a chance that diagnoses will become safer and faster – and all without the human factor. This is a topic in which Siemens is also interested.
One of the first applications we will offer with this IT platform is the optimisation of dosing protocols
Peter Aulbach
Currently, one of the main objectives is the development of a fundamental telecommunication base because comprehensive data processing necessitates feeding the machines with information. As a global manufacturer of medical technology, Siemens has access to masses of usable data. However, utilising this data requires the customers’ consent. Currently, the company is developing a cloud-based network entitled ‘Teamplay’, which not only is used but also ‘fed’ by doctors, clinicians and other healthcare providers.
Customer data is anonymised, collated and processed, based on certain patterns. ‘One of the first applications we will offer with this IT platform is the optimisation of dosing protocols,’ Peter Aulbach reports. ‘We derive and evaluate patterns from the dose values supplied by the users. We then replay the results to the customers. This enables larger hospitals, for instance, to check whether their CT scanners adhere to the threshold values in all locations.'
Even more interestingly, it also allows different centres to measure themselves against one another. If a hospital is hoping to be among the world leaders when it comes to curbing radiation doses it can use our system to check what it should do to keep up with the ten best centres in this field. ‘Quality assurance, such as found in the manufacturing industry for years, could then finally become reality in the healthcare sector as well,’ Aulback concluded.
23.02.2016