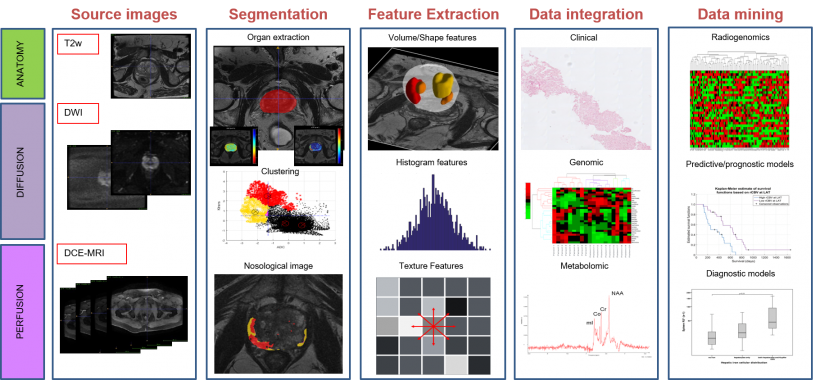
Deep learning-based: from feature extraction to data integration and data mining.
Source: Martí-Bonmatí
Article • Artificial Intelligence
Allying AI to biomarkers is powerful but validation remains challenging
Using artificial intelligence (AI) to push development of imaging biomarkers shows great promise to improve disease understanding. This alliance could be a game changer in healthcare but, to advance research, clinical validation and variability of results must be factored in, a prominent Spanish radiologist advises.
Report: Mélisande Rouger
In clinical practice efforts are already ongoing to apply AI to obtain new imaging data and improve the stepwise development of radiomics and validation of biomarkers, Professor Luis Martí-Bonmatí, Head of Medical Imaging at La Fe Polytechnics & University Hospital in Valencia, told delegates at the ESR AI Premium event last April in Barcelona. ‘There’s a mass of imaging data obtained daily and waiting to be deciphered and analysed to understand what’s happening in every patient across the huge diversity in people, diseases and disorders,’ he pointed out.
Using genomics, proteomics and metabolomics, scientists can already unveil biological processes in an individual patient. Computational medical imaging enables evaluation of tissue properties and behaviours from medical images, to accurately describe things relevant to a patient at a specific time. Using computerised modelling, mainly thorough deep learning techniques, high-dimensional data can be extracted and mined to build descriptive and predictive diagnostic tools. Highly performing computational techniques, such as Convolutional Neural Networks (CNN), help to provide new information on tissue expression diversity from ‘real world’ imaging studies, making a noticeable contribution to advancing healthcare. ‘If we can implement computer-based processes designed to analyse medical images to depict and classify those tissue changes, with their value and distribution, we might have a nice tool in our hands to improve personalised medicine using medical images,’ Martí-Bonmatí said.
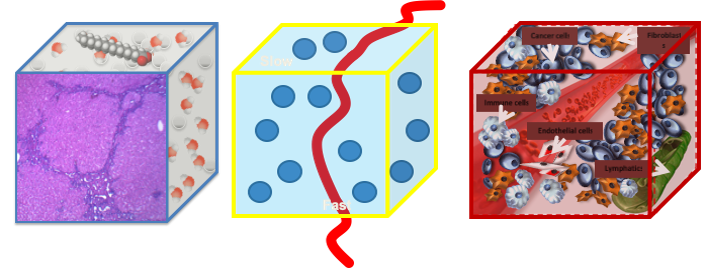
For any image measurement to be representative of a physical reality, it must have an unambiguous relationship with the process it measures.
Signal comes from voxels, which are complex structures in their components and properties, reconstructed from different machines.
Source: Martí-Bonmatí
The basics of biomarkers
Not every radiologist is familiar with biomarkers, yet they could transform radiology clinical routine and deeply impact on healthcare. Biomarkers are similar measures to those obtained from blood samples; they may indicate biological processes, pathological changes or pharmaceutical responses. When biomarkers are imaged, subrogated features and parameters can be obtained that will give quantitative information on regional distribution of these changes whenever necessary. In other words, tissue changes can be depicted over time and located, meaning they are resolved in both space and time. As images do not harm the organs and lesions, researchers can evaluate heterogeneous distribution of whatever they want to look at, whenever they want to look at it.
Biomarkers can be used to diagnose phenotyping, so to detect or confirm the presence of a disease, or to identify different diseases sub-types and even different habitats within a single lesion. They also can be used to assess why a tumour is responding to treatment, whilst other identical tumours are not, and to measure susceptibility to potentially develop a disease.
When linked to treatment effect, biomarkers can have predictive value not only on therapy effectiveness, but also safety, by looking at the extent of toxicity, a well-known adverse effect. ‘Ideally, we could also have biomarkers on prognosis and help determine likelihood of disease recurrence or progression, or patient survival, by taking a look at the lesions and organs where the abnormalities are present right at the beginning of treatment decision to predict which therapy will work best,’ he suggested.
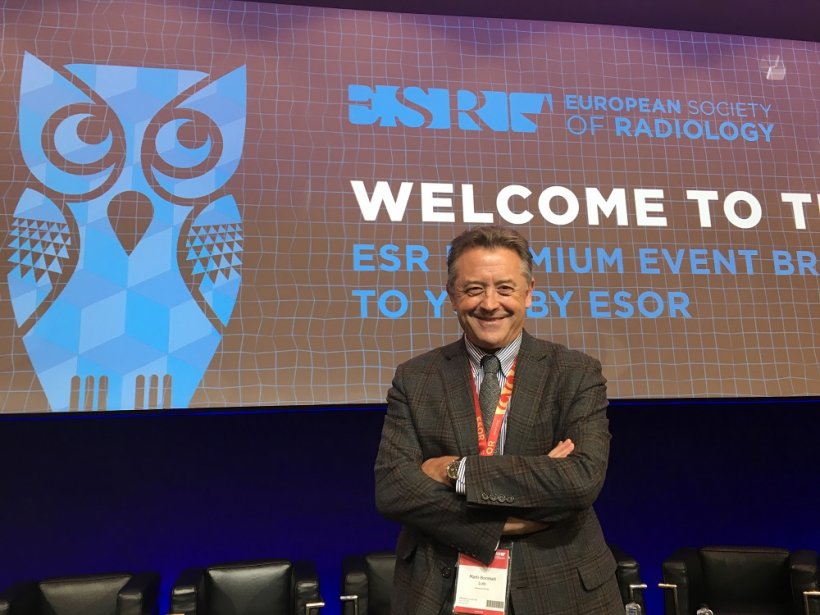
In daily practice, huge amounts of images are generated from different modalities and sequences, using a broad diversity of information channels for acquisition. Image preparation, including registration, analysis, resizing, intensity normalisation and tissue segmentation, are the next step. ‘We need to virtually ‘take out’ the organ or tumour we want to evaluate, to check what happens in that tissue as automatically as possible. Once we obtain this volume of interest,’ he said, ‘we can move to picture feature attributes, which is mainly radiomics, and go into morphology or semantics, spatial distribution of signal intensity, and histogram distribution, with all the different texture features that can be obtained across organs and tissues.’
Dynamic model parameters can also be used in the region or volume of interest, by calculating values that might describe what is happening in a given lesion through histograms, distribution statistics or spatial distribution of feature metrics.
A huge amount of data has been generated at this stage, so efforts must focus on reducing data, and then applying statistics or multivariate analysis or classifiers like clustering signatures. ‘We might be lucky and link whatever we have here with the diagnosis, predictive or prognostic endpoints that are our interests,’ Martí-Bonmatí said: ‘And then we will have biomarkers.’
Pending validation, profuse variability
Obtaining biomarkers is not an easy task and external validation in the clinical setting is key. ‘The relevance of those imaging findings and their correlation with the clinical endpoints and the impact on healthcare pathways must be shown,’ he pointed out. For example, in texture analysis of liver metastasis, some parameters might enable classification of lesions into those that will respond and those that won’t, right from the onset of treatment. ‘That’s quite good for us.’
But the big challenge remains future variability – unavoidable because image acquisition parameters still differ from one examination to another. ‘If we slightly change repetition time, echo time, flip angle, slice thickness on an MR sequence, the obtained parameters will change. Processing techniques, methods, filters, quantification levels and image depth will also change the radiomic features,’ he said. ‘So, small changes in so many variables will change the results.’
Signal dynamic parameters in MR techniques, such as intravoxel incoherent motion on diffusion-weighted sequences, may offer additional parameters that can be linked to aggressiveness of prostate cancer, for instance. But, the variability with this approach needs consideration – number, distribution and magnitude of the b-values, signal strength, amount of noise and lesion type will all impact on the calculated parameters.
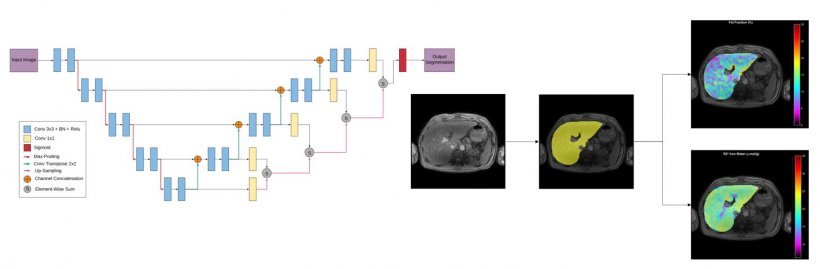
Liver automatic segmentation using deep supervision methods for whole liver fat and iron quantification.
Source: Martí-Bonmatí
Using voxel-enhanced dynamics, again in prostate cancer, the cellularity metrics obtained from the intravoxel incoherent motion, and the permeability Ktrans obtained through a pharmaco-kinetic model after contrast administration, may change widely in a clustered way. ‘If we perform a multivariate, multiparameter map, we can visualise those areas with high cellularity and high vascular permeability, which are the most aggressive ones. Unfortunately, the variability using these multivariate techniques together increases exponentially,’ he explained.
Therefore, researchers should ask themselves a number of questions: do they have the right and precise answers by using texture analysis or feature properties or parameters from signal dynamics? Will this process prove useful in clinical practice? Why are the best answers to the right questions still wrong? ‘Wrong means that we have a huge amount of variability in what we are doing. So, we have to look for the uncertainty of our truth. And we have to recognise that for anything we measure from images to be representative of a physical reality, we must have a clear relationship with the reality we are measuring. Imaging signal comes from voxels, and voxels have a huge amount of complex inner structures, all with different properties and components, obtained with different protocols, techniques, and machines. So it’s close to impossible to have a standardised image processing or image acquisition or parameters. Once we recognise this,’ he concluded, ‘we can work on ways to improve our work.’
Profile:
Luis Martí-Bonmatí MD PhD serves as Head of the Clinical Area of Medical Imaging at La Fe Polytechnics & University Hospital, Valencia, Spain, and a member of the Spanish National Royal Academy of Medicine. He is also the founder of QUIBIM S.L. and serves as its Director of Scientific Advisory Board.
29.05.2019