Article • Potentials and pitfalls for IB development
Imaging biomarkers: Close surveillance is mandatory
Imaging biomarkers (IB) have advanced tremendously since first described 25 years ago, but many challenges still block their widespread use. During the EuSoMII’s annual meeting in Valencia, Dr Ángel Alberich-Bayarri gave pragmatic solutions to tackle current bottlenecks and explained why close surveillance is mandatory for further development of IB.
Report: Mélisande Rouger
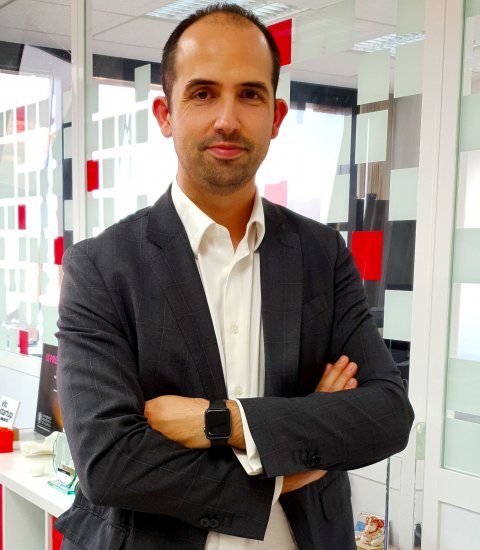
However, these need close surveillance with properly reported results. ‘New drugs need strict evaluation phases in the form of clinical trials and be approved by regulatory bodies to reach the market,’ he advised. ‘Even after being approved, a close surveillance of safety, effectiveness and efficacy is performed in a large number of patients,’ he pointed out. ‘We have to adopt a similar structure for IB and AI; if we are structured in therapy design and development, we also should be good in detection and diagnosis. Technical sheets of any drug specify where the drugs work better, or in which population they have not been tested.’
A solution could well be to use publicly accessible technical datasheets of algorithms to communicate their performance and errors in real-world data. ‘QUIBIM will showcase this approach at ECR 2020,’ Alberich-Bayarri said.
Reproducibility crisis
We must assume that sometimes we will not be able to measure accuracy because of the lack of ground truth
Angel Alberich-Bayarri
Having information on a measurement’s performance and failure could help to solve the major issue of reproducibility. ‘There’s a reproducibility crisis in imaging biomarkers analysis and AI algorithms. We use many tools from different resources, in different hospitals and environments,’ he added. ‘We don’t know which tool is best.’
Radiologists cannot evaluate even the simplest measurements currently available. They don’t have a single value on what Alberich-Bayarri called the ‘confidence interval’. In other words, they have no idea if a measurement is reliable or not. ‘This is quite surprising, because even for a simple thermometer, we will find the confidence or the uncertainty of measurements performed with that device. So, he pointed out, ‘We urgently need a methodology philosophy for imaging biomarkers.’
The EIBALL’s statement paper on the validation of biomarkers might be a proposal from which to draw inspiration. The validation pipeline is mainly focused on checking an algorithm’s precision, accuracy and clinical validity with the clinical outcomes.
Precision is easy to evaluate by looking at both the influence of biases at the image acquisition level (machine versions, manufacturers, parameters) and at the image analysis methodology level (algorithms and models used). Accuracy can be assessed by comparing a biomarker with solutions that have demonstrated their reliability, for example concentrations or different properties, and of course pathology, by comparing results of a measurement with a sample’s real value. ‘However, we must assume that sometimes we will not be able to measure accuracy because of the lack of ground truth,’ Alberich-Bayarri said. Clinical validity of an algorithm can be measured not only in the short term, by evaluating diagnostic and therapeutic problems, but also in the long term, i.e. finding biomarkers with a prognostic value.
Manual interaction with client-server solutions or workstations is a bottleneck for IB widespread use. A solution is to use a rules engine to execute a specific AI image analysis fragment and imaging biomarkers analysis pipeline. ‘In any case we have to keep humans in the loop. Even if the pipeline is completely automated, it must incorporate checkpoints for user interaction,’ he advised.
Computational resources have to be ready for massive analysis of imaging biomarkers, and one strategy is to design and implement high performance computing elastic infrastructures after the necessary stress tests, evaluating analysis times and reports delivery with a high number of cases to be analysed.
Recommended article
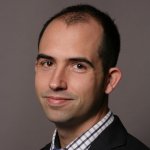
Article • Image analysis in radiology and pathology
"The time has come" for AI
AI has made an extraordinary qualitative jump, particularly in machine learning. This can help quantify imaging data to tremendously advance both pathology and radiology. At a recent meeting in Valencia, delegates glimpsed what quantitative tools can bring to medical imaging, as leading Spanish researcher Ángel Alberich-Bayarri from imaging biomarker company Quibim unveiled part of his work.
Structured reporting
There are several solutions to integrate IB with structured reporting (SR), which is another current difficulty. ‘We can combine AI algorithms, imaging biomarkers analysis and structured reporting in same environment,’ said Alberich-Bayarri, who has developed a CE marked architecture capable of hosting and integrating all these elements for prostate applications. The main goal is to avoid workflow interruption and to be prepared for massive analysis computing performance. ‘Using these types of architecture,’ he said, ‘our main aim is to convert our radiology department into a quantitative radiology department, where all biomarkers are seamlessly extracted.’
Radiologists will not manage to integrate SR with quantitative imaging if SR is independent from the user and biomarker’s interface. ‘Radiologists,’ he concluded, ‘want the SR integrated with AI and biomarkers. So we have to think of changing our current paradigm.’
Profile:
Ángel Alberich-Bayarri PhD is the CEO of QUIBIM, a company that applies AI and advanced computational models to radiological images to measure changes produced by a lesion or pharmacological treatment, offering quantitative information to radiology.
12.03.2020