Source: Pexels/Markus Spiske
Article • A valuable assistant
AI will transform radiologists into data scientists
Machine learning is increasingly helping radiologists to acquire faster and better quality images, and measure heart function. This is just the tip of the iceberg; artificial intelligence has far more to bring to the heart, explained Daniel Rueckert, Head of the Department of Computing at Imperial College London, during CMR 2018.
Report: Mélisande Rouger
Machine learning (ML) is becoming a valuable assistant for radiologists performing cardiovascular MRI. ML tools mainly help clinicians to acquire better images of the heart in shorter episodes of time, a key factor that can improve examination quality and increase patient comfort, according to Rueckert. ‘Typically, patients need to hold their breath several times while the examination is being performed. The sicker you are, the more complicated it is to hold your breath, and this may impact examination outcome. So here the machine simply helps you get the images faster and in a more comfortable way,’ he said.
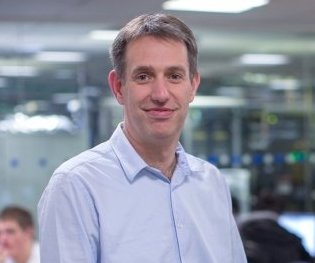
ML also enables functional parameters measurement, for instance of how much blood the heart can pump per heartbeat. To do that, radiologists typically measure the heart when fully relaxed and when contracted. The difference indicates how much blood the heart can pump. The operation requires labelling every pixel in the image depending on which part of the heart is being observed, so one can actually compute that measure; but with machine learning, this can now be done automatically, Rueckert said. ‘You can effectively analyse the images and automatically perform segmentation. Then you show the clinician what segmentation looks like, and if they’re happy, they can accept that and get these measures automatically.’
For the moment, machine learning use is limited to assisting the radiologist in doing ‘basic’ tasks. But this is just the bottom of the pyramid, said Rueckert, who used the reference to illustrate the machine’s contribution to medical imaging. ‘In the future ML may well help the radiologist to perform high-end tasks, for instance directly diagnose disease from an image. Research is underway in this field and applications are emerging, but it may be a while before they effectively serve the radiologist,’ he said. The solutions developed so far will not replace radiologists, but rather make things easier for them at work, he added. ‘A lot of what has been developed in recent years are techniques that would make working easier for the radiologist, but are not designed to replace the radiologist.’
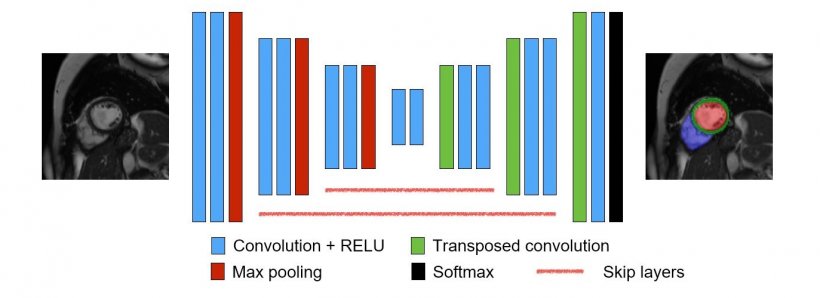
© Bai et al. 2018
Clinicians need to understand what is being said and to know what they can rely on; when should they trust the machine and when it is just an opinion
Daniel Rueckert
Rueckert’s comments followed debates about radiologists potentially losing their jobs to ML and other artificial intelligence-based solutions. This, Rueckert is sure, will definitely not happen. ‘ML is a tool which radiologists will all use in the future when they look at images, and it will help to make better decisions based on better images. But I repeat, it will not replace them,’ he said. Artificial intelligence has the potential to boost the radiologist’s contribution and value in the chain of healthcare – for example by bringing in quantitative assessment to the radiologist’s report, an objective information that is currently missing. ‘When radiologists look at the image and then make a report, they give you a very qualitative assessment of what they have seen. A blood test will give you a reading value, a quantitative measure, but an imaging examination won’t give you that for the moment. I think we will see much more quantitative radiology. The report will give you a number and that number might be a criteria of information, Rueckert suggested. This will help establish the probability for a diagnosis, and may help clinicians to diagnose earlier and more confidently. Quantitative measures may also help identify further conditions, which often go unnoticed when screening for a particular disease, Rueckert added. ‘One thing that is quite overlooked is that it’s quite common for people to have a multitude of diseases; you often have a mix of things. Taking in a probability for a certain disease may be the right thing to do, because it can reflect that you have certain other diseases,’ he said.
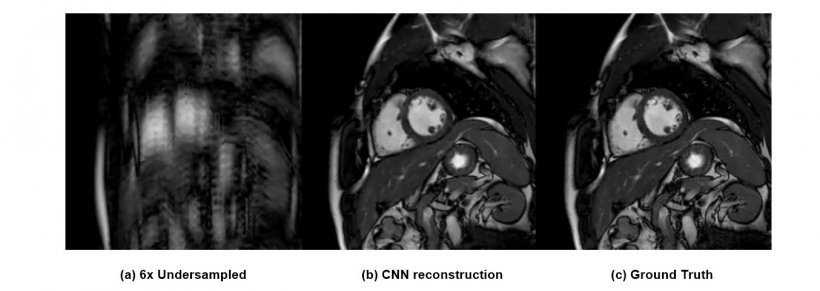
© Schlemper et al. IEEE TMI 2017
A myriad of potential applications could benefit from ML, not only risk identification and disease prediction, but also treatment guidance. ‘If you want to perform heart surgery you need imaging to guide you; so, ML could help use imaging more appropriately in this setting as well,’ he explained.
Machines may not threaten radiologists, but their jobs will be transformed. ‘ML will probably make life a little bit more complicated for radiologists, who in the future may become something more like data scientists – people who need to interpret a lot of information from different sources, from imaging and maybe from non-imaging examinations – genetics tests for instance. So, their work might become more complicated, but technology will support them in that change.’ Radiologists will need proper training to be able to use these tools, communicate with computer scientists and understand when to and not to trust the machine. ‘Clinicians need to understand what is being said and to know what they can rely on; when should they trust the machine and when it is just an opinion. The machine cannot give you a definite answer but it can help in case of doubts.’
Profile:
Professor Daniel Rueckert heads the department of computing at Imperial College London, which he joined as a lecturer in 1999 and became senior lecturer there in 2003. He has been professor of visual information processing and led the biomedical image analysis group since 2005.
01.09.2018