Image source: Shutterstock/Nadya C
Article • Transferring research into daily routine
AI possibilities and probabilities
Although some people foresee artificial intelligence (AI) easing medical workloads, many challenges arise before that dream can begin. Dr Felix Nensa and Dr Bram Stieltjes described such hurdles in an ‘Artificial Intelligence in Medical Imaging’ session held during a SITEM School Symposium in Bern, Switzerland.
Report: Daniela Zimmermann
Whilst AI has potential, actually delivering that asset into routine medical practice remains a major challenge.
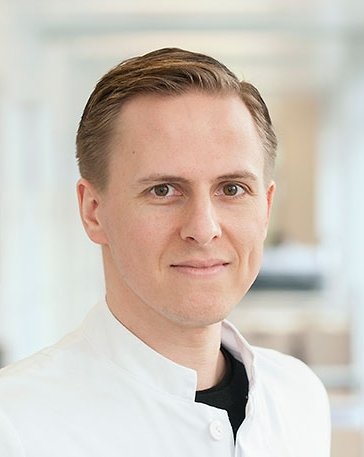
Recently discussing ‘Translational AI – from bits to bedside’, radiologist Dr Felix Nensa, from Essen University Hospital in Germany, pointed to headlines that AI had helped to save lives, or that machines were supposedly better than radiologists. But, he noted, the tone of those headlines has been changing – indicating a peak in AI development. ‘Now,’ he said, ‘to get to a plateau of productivity, we really need to be translational. We need AI to do something useful for us.’
The hype around AI results from several decades of continuous exponential growth in computer hardware, the power of computers, and storage, which brings with it a lot of data.
Medical informatics is needed to deploy AI tools into a hospital infrastructure because if it doesn’t work in the clinical routine, it’s worth nothing
Felix Nensa
At the centre for liver transplantation in Essen, he said a tool has been created to automatically segment the whole liver and produce reports. He also outlined how machine learning can be used to predict how liver lobe will grow and predict future liver remnant in patients. A good machine learning model starts from a good question, he emphasised. ‘If you identify a clinical problem find what really needs improvement and then understand the problem and specify data, you can communicate that with data scientists, who can design a system. After that, it’s important to get these clinical models back to clinical routine early, to validate them.’ While the first try-out will probably not work that well, the process is repeated with further iteration to deliver a tool good enough to work in clinical routine.
Nensa outlined the importance of medical informatics in translation and integrating tools into workflows. ‘Medical informatics is needed to deploy AI tools into a hospital infrastructure because if it doesn’t work in the clinical routine, it’s worth nothing,’ he added. In bone analysis, for example, AI could be used to mimic a radiologist’s workflow with stacked neural networks, harnessing the specialist’s knowledge of workflows into the data science for tools to work better.
However, Nensa stressed that he does not think AI could or would replace radiologists or radiology. ‘Radiologists using these tools will get much more efficient much faster than those who reject them. In the end, radiologists who refuse to use these tools will be replaced – not by AI, but by other radiologists who do use AI.’
Is big data always big value?
However, Nensa added: ‘You have to be careful not to equate big data with big value. Only if we have the ability to analyse this data does it turn into value. We are producing more information but lagging behind in our ability to analyse this data. The big hope is that AI, or methods of machine learning, will help us close this gap and extract more value from our data.’
Sharing examples from radiology, he said decades of information from images had simply gone into archives and nothing further had been done with that valuable data. However, radiomics – among new buzzwords in radiology – is seeing information extracted from tumours, in terms of their shape and texture, with machine learning applied to the data to create predictive models or future treatment plans.
A tumour’s N- or M-stage, he said, may be predicted by looking at a primary tumour. Or, for brain tumours, where there is reluctance to carry out a biopsy, machine learning on PET-MRI data could be used to predict its mutational status. Yet, he still does not consider radiomics translational, because most methods need a manual tumour segmentation, and there are also issues of standardisation, validation, and clinical acceptance. ‘Radiomics is interesting research-wise and we should go for it, but there is almost nothing I see in five to ten years in clinical routine,’ Nensa said.
Recommended article
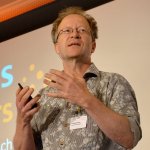
Article • AI in diagnostics
Learn like a human, deduce like a machine
Artificial Intelligence (AI) is like a huge blanket that can cover anything from innocuous chess computers to robots which, depending on your viewpoint, could save, oppress or obliterate humanity. However, not every jar labelled AI contains AI. So what is intelligence and can it be created artificially, synthesised like a nature-identical flavouring substance?
On AI use theories recently discussed at major radiology conferences, as well as the point about radiologists already operating at near capacity and needing new technology to become more effective, he said: ‘That’s because we are getting more data every day and we cannot scale by just getting more efficient by ourselves, so we need tools to help us to scale and provide real-time diagnostic support.’
Rather than an autopilot, AI will be a co-pilot and leave radiologists to focus on vital tasks. It will not take jobs from radiologists, he confirmed, but will aid efficiency with routine tasks delegated to automation. ‘In the end,’ Nensa concluded, ‘we’ll have super-diagnostics, where all this information will come together and we, with the help of machines, will have oversight of all data, providing better and more sophisticated real-time diagnostics. This is key to personalised medicine.’
Challenges to AI projects
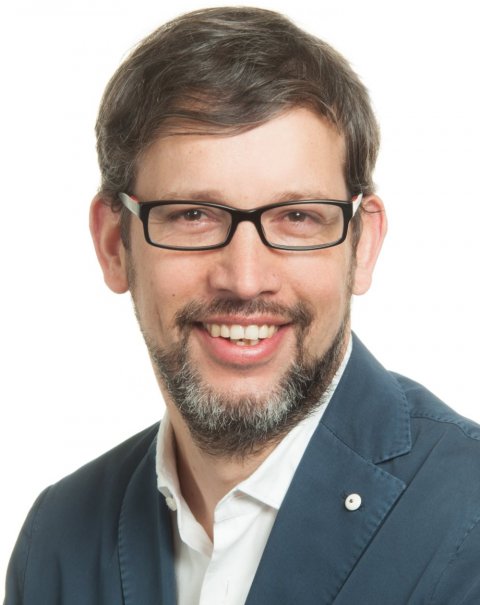
Dr Bram Stieltjes, from Basel University, outlined a range of challenges and hurdles involved in developing artificial intelligence projects in a clinical context. As Head of the university’s Research and Analysis Services Department, his presentation ‘AI in a clinical context; get ready to make your hands dirty’, tempered expectations and focused on why AI in a medical context can be so difficult.
He warned that a ‘huge over-enthusiasm’ is circulating around medical data on what can be done in the context of AI. A key issue with machine learning is having sufficient information and items in reports, in the first place, to be able to extract meaningful information. In a significant proportion of cases, Stieltjes feels reports are incomplete.
For structured reporting, he said, standard sentences must be a key element, but the aspect of ‘probability’ is often overlooked, and its lack is a limitation. ‘Probability is the only way for us to know what you are thinking and the only way to use that data afterwards for a machine learning or AI project. If you do not add probability you are lost,’ he observed. Adding probability brings an extra dimension to reports and could help in future learning and thinking. ‘Recording medical thinking like this not only helps to train algorithms but also to train doctors in a really quantitative way. The whole structuring of medical data not only touches on training algorithms to replace what doctors do, but also trains doctors in a completely different way.’
There should also be a direct connection to the image. ‘Today, what you have with AI projects is that you are really looking for a needle in a haystack at best and sometimes, in searching for a needle, you just find hay.’
If we can turn that thinking error around, I think we’ll see rapid progress. If we don’t, I think we’ll talk like this for decades
Bram Stieltjes
Within a healthcare setting, he said, clinical data is saved for clinical documentation without much consideration of research. ‘It’s relatively easy to find all the data points from one patient, but even the databases that control our clinical applications are not built to find all similar patients, or all patients with the same exams.’
To create an effective machine learning application, he explained that auxiliary tools are needed that incorporate a risk element and technical descriptions. Stieltjes’s team at Basel University reorganised their available data for research purposes and re-indexed technical exams in a re-think on the way they were dealing with data.
Using the search engine for as many patients as possible who fulfilled their criteria, they could investigate 12,000 MRI scans in 48 hours. ‘It’s a whole different way to work and enables interactive data interrogation and maybe drives the hospital infrastructure,’ he said.
In a healthcare setting, he warned, there can be barriers to sharing data and acquiring it from other departments for an AI project, and also pointed to disappointments in the length of time it takes to develop an AI project. Moving radiology –and other fields – to structured text has intrinsic limitations, he said. Obtaining good data will still not solve all the problems, especially the connection between text and image.
However, in a scenario where all data created in a medical context is structured can help with modelling the data, and in making a virtual model of organs and predictions. ‘I think this highly depends on how seriously we take the data-driven organisation of medicine,’ Stieltjes said. ‘We shouldn’t organise ourselves around diseases, doctors and specialties; we should analyse what data elements are important for decision-making and how we get them.’ The focus should be more on the data stream, rather than what is necessarily most convenient for the clinician, he said. ‘If we can turn that thinking error around, I think we’ll see rapid progress. If we don’t, I think we’ll talk like this for decades.’
Profiles:
Directly after completing his final school exams, Felix Nensa freelanced as a software developer for the Research Institute for the Diagnosis and Treatment of Early Lung Cancer at the Augusta Hospital in Bochum. At that time he also took a distance learning degree course in IT (2000). His medical studies, at Ruhr University in Bochum (2001-2007), included two semesters at the University of Strasbourg in France. In 2011, Nensa became a registrar at the Institute for Diagnostic and Interventional Radiology and Neuroradiology at Essen University Hospital. He manages research projects on PET/MR, Cardio-MRI and DCEMRI.
Bram Stieltjes MD PhD is VC of Research at the Department of Radiology of the University Hospital Basel and has recently formed a new department for research and analytical services in IT. In this role, he aims to integrate technical developments in physics and computer science into medical data science projects. His resident training is in radiology but he also had a year of training in child psychiatry and radiation oncology. He also headed a multidisciplinary group consisting of members from physics, medicine and informatics at the German Cancer Research Centre.
05.11.2019