Article • USA and Chinese experts share observations
AI in Covid research
A panel of experts from the USA and China highlighted AI use in radiological workflow during the Covid pandemic and identified current pitfalls during the Hot Topic session at RSNA 2020.
Report: Mélisande Rouger
A radiologist’s experience in the US
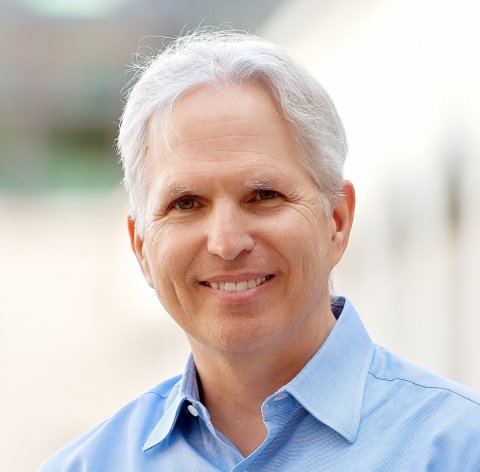
Radiologists from the USA prioritised Covid articles, delivered quick reviews, made all results open access, and helped organise a white paper from the Fleischner Society recognising recommendations for the role of imaging in the disease. A multitude of expert webinars and studies were released, e.g. ACR guidelines on safe resumption of non-urgent radiology care.
With Covid, diagnosis is the first necessity an AI tool should fill in medical imaging, according to Greg Zaharchuk, Professor of Radiology at Stanford University. ‘This was important in the beginning of the pandemic, when systems were overwhelmed and when there was lack of access to point-of-care gold standard tests. It was,’ he added, ‘also useful to have an AI tool when radiologists were not familiar with the disease.’
Another need is to predict disease course and outcomes, including disease severity, length of hospital stay and requirements for ICU and ventilators. It would also help to predict the appropriateness of certain treatments, e.g. need for anticoagulant therapy, and to have an automated system to predict a patient’s trajectory.
Standardised reporting
CT enables quick acquisition and reading – very useful when gold standard viral tests are not available, Zaharchuk suggested. ‘CT has a characteristic pattern with these peripheral ground glass opacities, mostly in the lower lobes. It can be done with low dose at .5 mSv technique.’ But he pointed out issues with cleaning, access to machines and personnel exposure. ‘It would be useful to have a system in which we could all try to agree on what constitutes CT findings with different probabilities of Covid. Standardisation is quite important for AI algorithms.’
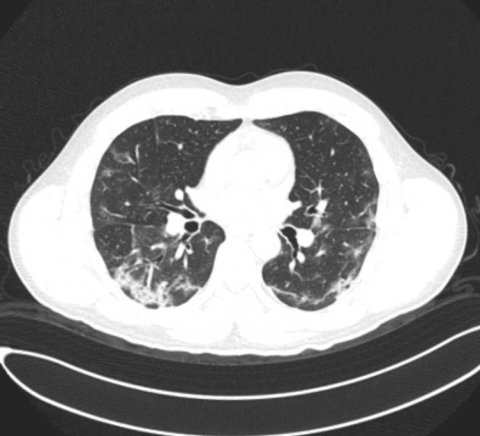
In a collaborative project, Mount Sinai, MGH and several Chinese hospitals looked at 905 patients, in whom they evaluated a combined approach: looking at CT slices using a CNN model and including non-imaging information, such as lab tests. ‘Three models were developed and the combined system tended to work best. AI models showed 0.92 AUC and had very similar performance to radiologists,’ Zaharchuk said.
Another study by an international group including researchers from MGH, NIH and NVIDIA took a very conventional approach and used segmentation of the lung using either a full 3-D model or hybrid 2-D model that was patch based to a DenseNet, to predict the presence or absence of Covid pneumonia. ‘Their prediction was very similar, with AUC of around .9, and sensitivity around 80% and specificity around 90%,’ he said.
Recommended article
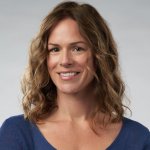
Article • Open source framework
Here comes the AI healthcare era
Although the Covid-19 pandemic has put wind behind the sails of AI in healthcare, domain specific tools are needed to build and deploy AI and harness its power in data handling, training workflows and reproducibility of state-of-the-art approaches, according to Kimberly Powell, NVIDIA Vice President of Healthcare at the technology firm NVIDIA, presenter of a public address at RSNA 2020.
Chest X-rays have characteristic patterns but can be more challenging to interpret. Acquired quickly, they are useful in a setting where gold standards are not available, Zaharchuk said. Machine cleaning and exposure to personnel are lesser issues. Thus AI studies have been performed with large numbers.’
Covid is a multi-system disease but we have very little data outside the lungs so far. Future randomised trials might be very helpful to help us individualise care
Greg Zaharchuk
In many studies algorithms developed for Covid diagnosis with X-ray showed good or excellent AUC, similar to, or even exceeding, thoracic radiologists’ performance. Predicting severity of disease is another advance that AI must bring to Covid management. Early literature showed that existing software could be repurposed to identify ground glass opacities, do segmentation and, using a slice-based method, predict the severity of Covid abnormalities. ‘(Authors) proposed the use of a corona score, i.e. the volume of abnormal tissues in the lung, and demonstrated the score could be used for Covid. But you can imagine it could (also) be used for any viral pneumonia or any pneumonia,” Zaharchuk said.
Another MGH group approach focused on the use of Siamese neural networks, frequently used in patient facial recognition, when looking and describing similarities. The researchers used pre-training on the CheXpert dataset to try to identify normal from abnormal datasets and give a pulmonary X-ray severity score, with good results.
All studies showed very high value of transfer learning to then fine tune with a small number of Covid positive cases, Zaharchuk said. However, AI research must look beyond the chest for information to really make a difference. ‘Covid is a multi-system disease but we have very little data outside the lungs so far. Future randomised trials might be very helpful to help us individualise care,” he concluded.
A view from China
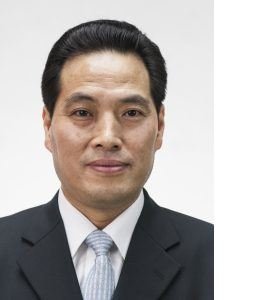
China’s National Health Commission issued eight editions of diagnosis and treatment guidelines that played a decisive role for pandemic control, explained Shi Yuan Liu, Professor of Radiology at ChangZheng University in Shanghai. ‘According to this commission,’ he said, ‘medical imaging plays an indispensable role throughout whole process of diagnosis and treatment, including detection of lesions, confirmation of suspected patients, clinical classification, differential diagnosis, and hospital discharge criteria, especially in the outburst earlier this year.’
More than 3,270 Covid-related radiological papers were published this year, 69% of them using CT and then X-ray, ultrasound and MR; China contributed with 26% of the literature. Five Chinese companies, including traditional medical imaging vendors, claim they have AI pneumonia models, while research institutes develop and use their own AI models in clinical practice. Their first role in the pandemic control is to optimise workflow and facilitate infection control, Shi Yuan Liu explained. ‘AI helps radiologists work more efficiently. Data from Shanghai Public Health Clinical Centre (SPHCC) showed that AI models can significantly reduce manual examination time and AI specificity and sensitivity for detection can be as high as 97%,’ Zaharchuk said.
A second role for algorithms is in early detection and diagnosis of Covid, especially regarding differential diagnosis. Zaharchuk: ‘Differential sensitivity and specificity between Covid-19 pneumonia and community-acquired pneumonia is about 90%.’ For classification, AI-based quantification is very important in both diagnosis and treatment of new coronavirus pneumonia. ‘Quantitative information can probably include total volume and density of the lesion, volume and density of lung and quantification of pneumonia lesion.’
Follow-up
AI can also help dynamically observe the changes of the lesions. Although studies support AI use in medical imaging, there are shortcomings, especially regarding training data, which remains insufficient, Liu insisted. Perfect models should combine all the information, including clinical information and lab tests, and, in future, should be ‘whole information models’. Development of pneumonia AI models should have clear R&D objectives on their final output, to totally fulfil clinical scenarios and not only solve one side of a problem, Liu concluded.
Berkham Sahiner PhD, Senior Biomedical Research Scientist at US FDA also participated in the session, moderated by Jerome Z. Liang, Professor of Radiology, Biomedical Engineering, Electric and Computer Engineering, and Computer Science at Renaissance University.
Profiles:
Professor and Chairman of the Department of Radiology in the ChangZheng University Hospital in Shanghai, China, Shiyuan Liu has specialised in chest radiology, especially lung cancer screening and clinical imaging diagnosis for over 30 years. He is President-Elect of the Chinese Society of Radiology and of the Asian Society of Chest Radiology, and President of the Chinese Medical Imaging AI Innovation Alliance, and heads national AI research and applications in radiology. He received c. $6 million research grants from Chinese National Science Foundation, Ministry of Science and Technology, and Shanghai Local Research Foundation. He edits the Oncoradiology journal; has published 321+ journal papers and authored nine books.
Professor of Radiology at Stanford University and a neuroradiologist at Stanford Hospital, USA, Greg Zaharchuk is President-Elect of the American Society of Functional Neuroradiology, Deputy Editor of the Journal of Magnetic Resonance Imaging and sits on the editorial board of Radiology. He is also on the Scientific Program Committee, the Neuroradiology/Head & Neck Subcommittee of the Radiological Society of North America (RSNA), and is a Fellow of the American Institute for Medical and Biological Engineering (AIMBE). In 2016, he received the Distinguished Investigator Award of The Academy for Radiology & Biomedical Imaging Research.
03.12.2020