Source: Shutterstock/Lightspring
Article • AI ethics and responsibilities
A journey into human/machine interactions in healthcare
With Artificial Intelligence (AI) able to deliver diagnostic advances for clinicians and patients, the focus has shifted towards ensuring the technology is used in an ethical and responsible way.
Report: Mark Nicholls
As evidence emerges of a gap in research on ethical deployment of AI, Dr Gopal Ramchurn is embarking on a three-year research project that will look at setting parameters for AI usage, with a key aspect exploring the team-working approach between humans and machines and a methodology for those interactions.
When the AI gets it wrong, who do you attribute the fault to: the designer of the machine, the doctor who interpreted the data or the machine itself?
‘In terms of responsible AI – with software that has a human level reasoning ability – you need to be able to endow that with some level of understanding of what humans care about,’ he said. ‘Humans care about privacy, the meaningful use of their data and about the right choices being made on their behalf, but they also care about having control over how these choices are being made. So when you design AI that is ethical and responsible you need to factor in these aspects.’
AI expert Ramchurn, an Associate Professor in the Agents, Interaction, and Complexity Group (see profile), suggests that our understanding of these issues is currently limited. ‘Therefore, it’s very hard to define a standard set of principles that would help us ensure that all these levels of quality assurance are ethical and responsible,’ he said.
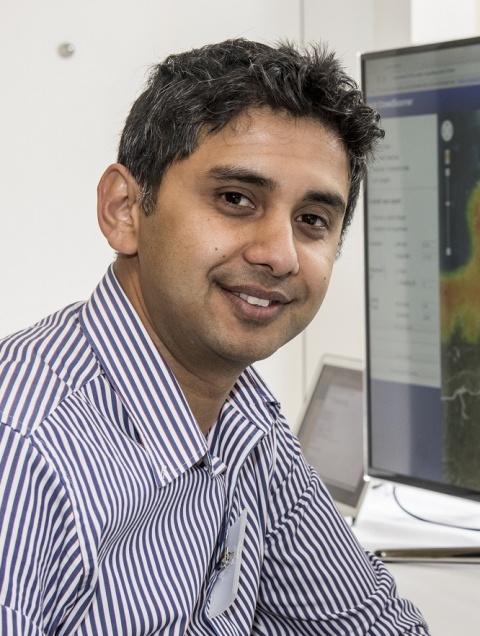
Funded by a €250,000 grant from the AXA Research Fund to investigate how AI can be responsible and accountable, Ramchurn’s research will focus on areas such as team-working between AI and human decision-making; where AI is deployed to support human decision-making; the extent that machine learning is deployed to make sense of insights for human decision-makers; and where computer vision algorithms identify particular features in support of human decision-making. ‘There are many instances of human/machine teaming,’ he explained. ‘That involves some level of coordination where the human has a goal and the machine has its own goals and has to interpret what the human wants, and the other way round.
‘In my new project we will look at how we develop methodology to design these interactions between humans and machines, deriving basic principles that ensure good human/machine understanding, interaction and goal setting and then establishing these design guidelines but also verifying that this methodology actually works. Intonation or language is very hard to understand for a machine so we need to have interaction designed – whether voice, text or app based – to engender some kind of trust between the human and the machine.’ This becomes even more critical in a healthcare setting, he said, with a need to build in safeguards in the form of explanations for why a machine is making certain decisions.
AI has already shown its proficiency in detecting cancers or heart disease, often better than doctors can, but he added: ‘When it gets it wrong – even 4% of the time compared to doctors who get it 20% wrong – who do you attribute the fault to: the designer of the machine, the doctor who interpreted the data from the machine or the machine itself?’ Data used for the AI approach also needs patient’s consent, and should be handled securely, he stressed.
Handling the control issue
Modelling risks around human/machine intelligence is a completely new topic
Gopal Ramchurn
‘My goal is to develop some of the underpinning technology that will ensure AI remains safe and responsible,’ he explained. ‘Some of the targets will be to look at how we can design AI systems to cope with varying degrees of user understanding and how we can design interactions with AI to make sure that control is given to users when it most matters, while the complexity of decision-making is dealt with by the AI when the user does not need to be involved.’ He remains concerned that medical community use of AI is still not always considering the risks. ‘Healthcare involves human decision-making outside machine decision-making,’ added Dr Ramchurn. ‘Modelling risks around human/machine intelligence is a completely new topic and that is why we need these new methodologies to define risks.’
He will also examine how such technology can bridge the gap between health and social care – from the hospital to residential care homes and linking and sharing data held by the hospital, family doctor, and care home.
Ramchurn’s research has centred on the development of intelligent software and robotic agents and how they work alongside humans and other agents. This next step will focus on the design of interactions with AI that ensures that humans have reasonable expectations about the behaviour of intelligent agents’. ‘We are at a critical point in time,’ he concluded, ‘where key questions are being asked about how artificial intelligence will change people’s lives – for better or worse.’
Profile:
Dr Gopal Ramchurn is an Associate Professor at the Agents, Interaction, and Complexity Group (AIC), in the Department of Electronics and Computer Science, University of Southampton. He is also director of the newly created Centre for Machine Intelligence, and Chief Scientist for AI start-up North Star. His research interests lie in the development of autonomous agents and multi-agent systems and their application to Cyber Physical Systems (CPS) such as smart energy systems, the Internet of Things (IoT) and disaster response.
28.11.2018