Article • Disease Research
Falsified genetic pathways may explain poor results
Spanish researchers are challenging the validity of many past and ongoing clinical trials and stress the importance of working with raw or preprocessed data in genetic information study.
Report: Mélisande Rouger
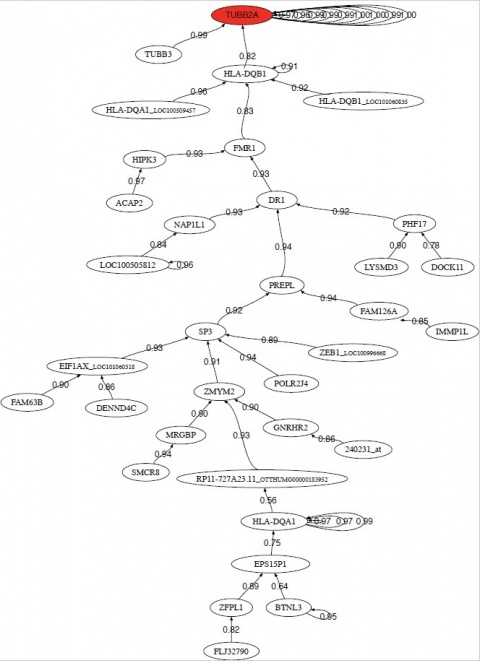
Preprocessed data, which are typically used to decipher gene expression in a large number of studies, modified and sometimes totally falsified genetic pathways in countless scenarios, according to a research team at Oviedo University, Spain, in collaboration with the Brigham and Women’s Hospital in Boston and NIH in Washington, USA. The results may have a tremendous impact on the understanding and prediction of many diseases, including cancer and rare and neurodegenerative disorders.
Professor Juan Luis Fernández, head of the group of inverse problems, optimisation and machine learning in the Mathematics Department at Oviedo University, was working on chronic fatigue in patients with prostate cancer undergoing radiotherapy when he found some discrepancy between results from the same measurements. ‘We found those results quite by chance when looking at genetic pathways before treatment, to detect which patients were more at risk of developing those symptoms,’ he said. ‘We published our first results in 2014, but a year later, when carrying out the same investigation with another data set, we obtained completely different results.’Upset but intrigued, Fernández and his team began to search for where this difference arose. ‘That was very disappointing; what we had originally done wasn’t bad and there we ended up with very different results. That’s when we noticed we’d been working with preprocessed data the second time. So we started processing raw data and realised the data we obtained was very different.’
Raw or preprocessed data?
Now enthralled, the researchers subsequently launched an investigation to find out which data was more accurate: raw or preprocessed? Preprocessing has the benefit of reducing the size of huge genetic data, which in turn makes data analysis easier to handle. To process genetic data, researchers most commonly use RMA (Robust Mean Average), a technique that enables noise reduction during data evaluation and a boost to the signal.
Working with raw data, Fernández and his team found they obtained less precise results in prediction. However, when working with preprocessed data, they could not locate the probes as reliably. While RMA enables amplification of signal-to-noise ratio, it also modifies genetic pathways. So, in the end, it is quite challenging to know which dataset is better to use, Fernández pointed out. ‘It’s almost a philosophical problem; if you mix up signal with noise, you interpret part of the noise as signal - and part of the signal as noise.’
Genetic pathways deduced from preprocessed data are different to those deduced with raw data. But, with raw data, one would coincide more with real biology than with preprocessed data, according to the engineer. A lot of researchers are only working with preprocessed data, but he believes there should be more studies based on raw data: ‘In principle it’s more convenient to work with raw data than preprocessed data when looking for genetic pathways.’
Currently, many genes are considered as therapy targets when possibly they are not even involved in the disease process. ‘Preprocessing may totally or partially falsify genetic pathways involved in disease development. This has serious impact on therapy targets investigation because, if you are looking in the wrong direction, your treatment or diagnosis will be mistaken.’
Current research is failing
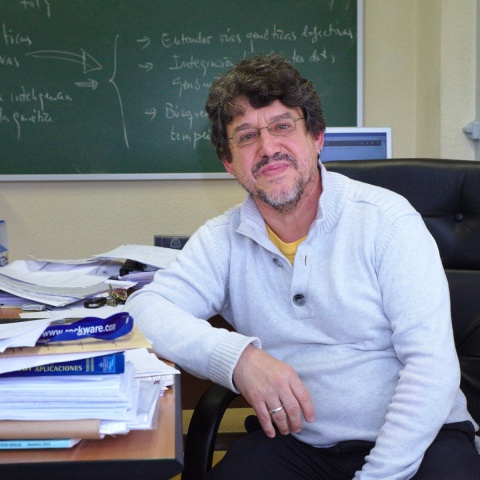
Fernández believes the absence of results in research speaks for itself. ‘Hundreds of billions of dollars are poured into disease research, and, to be honest, advances are very mediocre. So we must be doing something wrong.’
Furthermore, inadequately cataloguing samples in biomedical data analysis in expression pathways could have devastating consequences. ‘The worst case scenario is a physician doing false predictions and, for instance, telling his or her patients they won’t have metastasis – and then they do, all of this due to an error in genetic pathways analysis.’ Fernández said he had already obtained impacting results in pancreatic cancer and multiple sclerosis. He is finishing his work on inclusion body myositis (IBM), an inflammatory muscle disease characterised by slowly progressive weakness and wasting of both distal and proximal muscles. ‘We have demonstrated which genetic pathways are defective and, with a set of independent data, which pathways can fully predict phenotype. We have showed that the most important pathway is linked with viral and bacterial infection types, and we have possibly identified which infections.’
He said more results would soon be available in rare and neurodegenerative diseases. ‘With our analysis techniques we are able to validate blindly. We take an independent database and select the genes, then we take another dataset and get it right in almost 100% of cases.’
Raw data, rather than preprocessed, will offer more
Scientists must now urgently reach a consensus on which pathways they must consider in which scenario, knowing that raw data will probably give more information than preprocessed data, he added. ‘We have to perform retrospective analysis and be coherent. We may use raw or preprocessed data or both, depending on the situation. But we have to decide now. Some of the data you will obtain will completely change your understanding of disease.’
Profile:
Trained as a petroleum engineer in Paris (1988) and London (1989), and following years as an IT software engineer in France, in 1994 Juan Luis Fernández-Martínez gained a PhD in mining engineering from the University of Oviedo, in Spain. He joined the university’s mathematics department and became Professor of applied mathematics. During 2008-2010 he was a visiting and research professor at UC Berkeley-Lawrence Berkeley Laboratories and Stanford University. His expertise includes cooperative global optimisation methods, with applications in oil and gas, biometry, finance and biomedicine – in which he aims to design biomedical robots in translational medicine for diagnosis, prognosis, and treatment optimisation. The Finisterrae project particularly aims to find effective solutions for rare and neurodegenerative diseases, as well as various types of cancer.
16.05.2017