Article • MR Fingerprinting
Radiologists will become computer technicians
‘Magnetic resonance imaging is a very dynamic field,’ declared Professor Siegfried Trattnig, head of the Centre of Excellence for High Field MRI in the Department of Biomedical Imaging and Image-guided Therapy, at Vienna Medical University. Indeed, this September, two mega trends emphasised by Trattnig – the shift from qualitative to quantitative imaging and Big Data – dominated the 33rd Annual Scientific Meeting of the European Society for Magnetic Resonance in Medicine and Biology (ESMRMB) in Vienna, for which he is Local Organising Committee Chair.
Report: Michael Krassnitzer
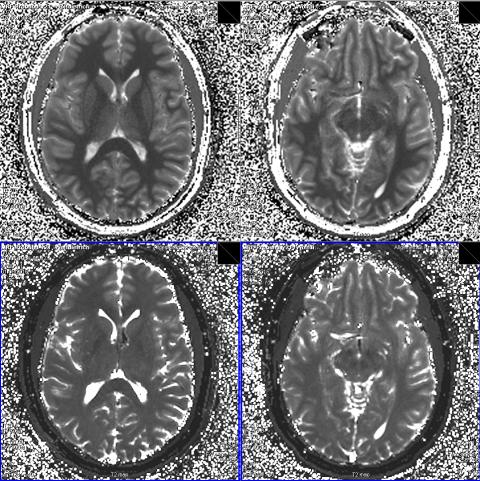
On conventional MR images lightness and darkness don’t correspond with absolute values but merely serve to help the radiologist make a diagnosis based on the respective contrasts. Modern MRI procedures, such as MR fingerprinting, however, can precisely quantify the three basic MRI parameters (T1 relaxation timings, T2 relaxation timings and proton density). This data can be used to generate maps which, at first glance, look like conventional contrast images, but which are capable of far more: they contain accurate measurements. Each pixel of a T1 map for instance corresponds with an exact T1 measurement. ‘This way the differences between pathological and healthy tissue can be clearly established. When monitoring the progress of treatment, for instance, it is possible to see how the measurements change, and to assess the efficiency of the treatment,’ Trattnig explains.
Until recently, the generation of a T1 or T2 map took about 10 to 15 minutes – too long for clinical routine applications. The new technologies make T1 or T2 mapping possible in one to two minutes. Furthermore, there is now the respective software that evaluates the maps at high speed. ‘This makes it really interesting for clinical routine,’ he points out. As the maps always contain the entire MRI information the conventional contrast images can be synthetically generated retrospectively. ‘There is no need to examine the patient with different sequences. A one-off measurement of the T1 and T2 values suffices,’ Trattnig explains.
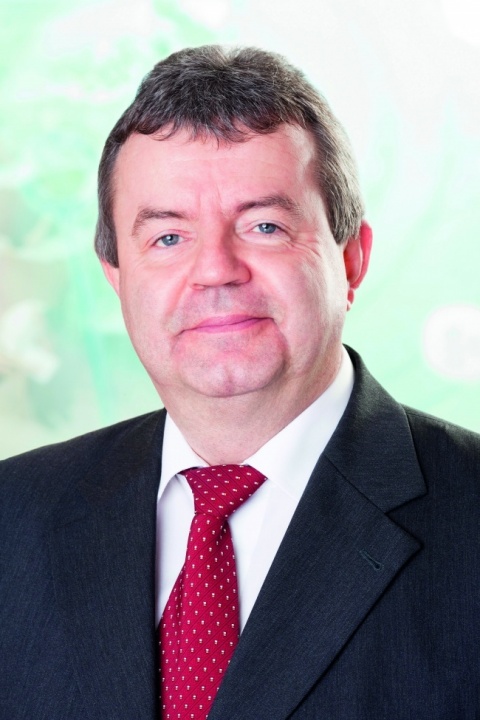
All these examinations generate vast amounts of data. As a representative from the industry reported at the ESMRMB Congress, Siemens alone has so many MRI scanners in use that 33,300 patients are being examined per hour worldwide. ‘If you have the respective tools, there is the opportunity to extract diagnostic information from this vast pool of data, which you cannot gain from individual examinations alone,’ says Trattnig.
Software based on machine learning can discover whether certain image data point towards certain diseases; it can discover correlations as yet unknown, or confirm suspected correlations respectively by analysing the large amounts of data.
Trattnig also expects the emergence of new findings from the comparison of imaging data with data from genomics, proteomics or metabolomics.
Big Data for instance is also essential for the ever more important research into dementia, he explains. ‘We need a very large control group to classify the normal aging process and to differentiate it from the early stages of dementia.’
These developments will lead to changes in practice for radiologists. ‘The radiologist will no longer make a diagnosis in the conventional way by standing in front of contrast images but will become more like a computer technician,’ Trattnig believes. Radiologists will have to acquire in-depth knowledge of the new procedures to keep up with the developments ahead.
Profile:
Siegfried Trattnig is professor for radiology with a particular focus on High Field MRI at the Vienna’s Medical University, where he has been Medical Director of the High Field MRI research scanner since 2000 and also heads the High Field MRI Centre (HFMRC) since its foundation in 2003. The professor is a member of over 50 committees in all important international, radiological, orthopaedic and MRI societies. Since its formation two years ago Trattnig has also been chaired the European Imaging Biomarker Alliance (EIBALL).
28.11.2016