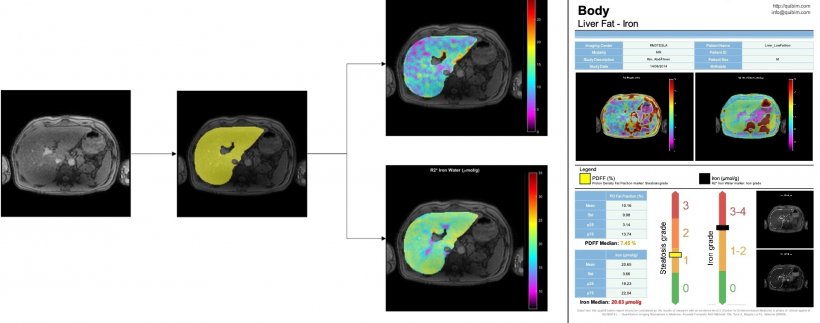
Article • Image analysis in radiology and pathology
"The time has come" for AI
AI has made an extraordinary qualitative jump, particularly in machine learning. This can help quantify imaging data to tremendously advance both pathology and radiology.
Report: Mélisande Rouger
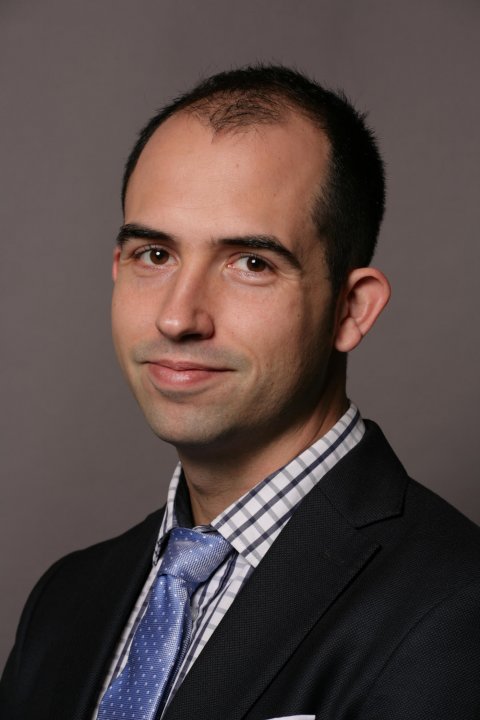
At a recent meeting in Valencia, delegates glimpsed what quantitative tools can bring to medical imaging, as leading Spanish researcher Ángel Alberich-Bayarri unveiled part of his work. The boom in companies and start-ups developing AI for tasks that impact on healthcare, and particularly pathology and radiology, is not about to fade. However, many have not looked at this potential properly, according to Alberich-Bayarri, scientific-technical director of the Biomedical Imaging Research Group (GIBI230), speaking during the Triangle meeting in January. ‘The time has come to discuss these things properly,’ he said. Alberich is CEO of Quibim, a spin-off company of La Fe Polytechnics University Hospital. The group develops machine learning tools to quantify imaging data, most notably a platform for quantitative image analysis and structured reporting capabilities, which has just received CE Mark certification as class IIa Medical Device for imaging biomarker analysis algorithms, zero footprint DICOM viewer and platform hosting these components and medical imaging data.
The four pillars of radiology acceleration
‘Quantification of imaging data is a sector that has a very high potential in clinical research studies, because AI, in this setting, can be used to speed things up considerably,’ he explained. Quibim works to accelerate workflows in pathology and radiology along four main axes: image reconstruction, segmentation, detection and data mining.
AI could help significantly to reduce acquisition times, for example in MRI examinations, by using raw data generated by the imaging modalities. Image reconstruction is currently the main focus of investigation, and Alberich works on algorithms that process data using deep learning for under-sampled MRI reconstruction. ‘Our aim is to identify all these regions, tissues and their potential variability. It would be a great advance,’ he said. The research community is experiencing a new paradigm, in which they receive all sorts of imaging data and aim to extract characteristics on shape, volume, texture, diffusion, etc. ‘We need to integrate all the data to be able to extract and mine information that we don’t know yet, to discover, for instance, new biomarkers giving an answer to unsolved clinical questions.’
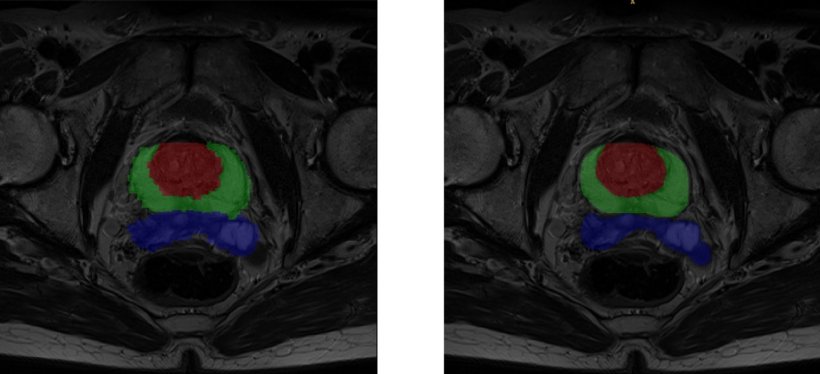
A game-changing new neural network for segmentation
One of the bottlenecks right now is segmentation, but this brand new area will greatly benefit from AI, Alberich believes. ‘Many engineers choose to subspecialise in segmentation right now, compared with, say, image registration because, as a new field emerges, new questions are raised and there is room for improvement,’ he said. In coming years, every work process including medical imaging will have to implement AI based segmentation because it will help save large amounts of time.
The U-net, a convolutional neural network that was developed for biomedical image segmentation at the Computer Science Department of the University of Freiburg, Germany, has been a real game-changer in segmentation and more generally radiology, Alberich explained. ‘The network’s modified architecture elegantly outperforms its predecessor, the sliding-window convolutional network,’ he said, ‘and the U-net can work with fewer training images, yet yield more precise segmentations,’ Quibim also uses deep supervision to generate output segmentation masks combining multi-layer and multi-resolution information. ‘Most of the critics on AI now target so-called ‘black box’ solutions, with one entry point and one exit point. So, an interesting line of work for us now is to get as much information as possible on the network’s dark layers to improve results and make the tool more understandable,’ Alberich explained.
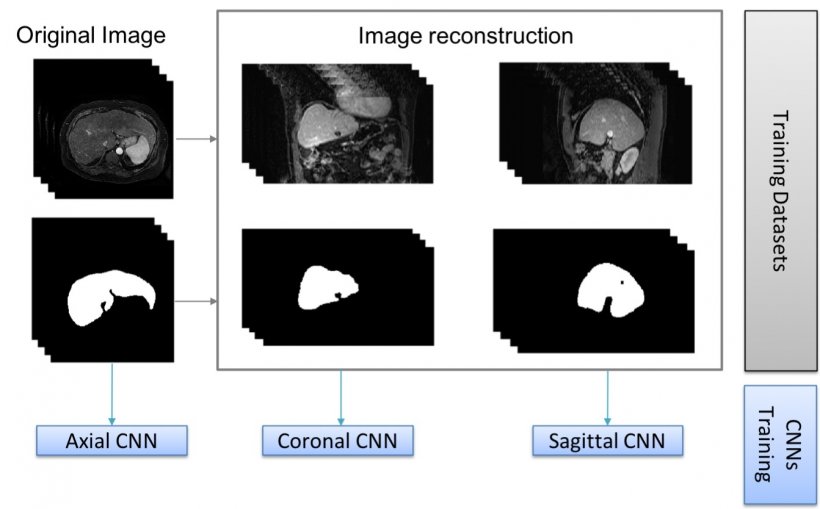
More perspectives lead to better insights
Segmentation techniques have already improved cartilage diagnosis, a service that was long confined to few specialised centres. ‘It used to take a biomedical engineer hours to segment the cartilage manually, and then parcel and calculate its properties,’ he pointed out. ‘Now the whole process is much faster and similar to virtual arthroscopy, which can be of value to orthopaedic surgeons. So we’re very interested in this potential.’ Alberich and his colleagues are notably working to label images in all planes, to improve results. ‘There may be errors in the liver when using only one network, which has been trained with either transversal, sagittal or coronal images. But when we combine all the information and generate a tissue probability map, liver segmentation is almost perfect,’ he explained.
In detection, once the structure and organs are visualised, it can be interesting for the pathologist to use clustering techniques – either supervised or non-supervised AI clustering. Both techniques can be useful, depending on the application. Non-supervised AI clustering can for example help extract new quantitative information and acquire more knowledge. The human mind is unable to visually study patterns in patient variation over time, and to group patients based on these criteria. Agglomerative hierarchical clustering could help in this regard, by helping to evaluate response. ‘Non-supervised AI can help unveil and extract information that we are not yet able to see on imaging,’ he added. ‘It can be very useful in these patients. Working with a set of variables is very good for recurrence prognosis in baseline diagnostic studies.’
Profile:
Biomedical engineer Ángel Alberich-Bayarri PhD is scientific-technical director of the Biomedical Imaging Research Group (GIBI230) at La Fe Polytechnics University Hospital and CEO of the spin-off Quibim, in Valencia, Spain. He is board member of the European Society of Medical Imaging Informatics (EUSOMII) and of the European Imaging Biomarkers Alliance (EIBALL). With over 10 years of experience in the development of quantitative image analysis solutions and their integration in clinical practice, he has co-authored more than 50 research papers and participated in more than 10 international medical imaging research projects.
26.02.2019