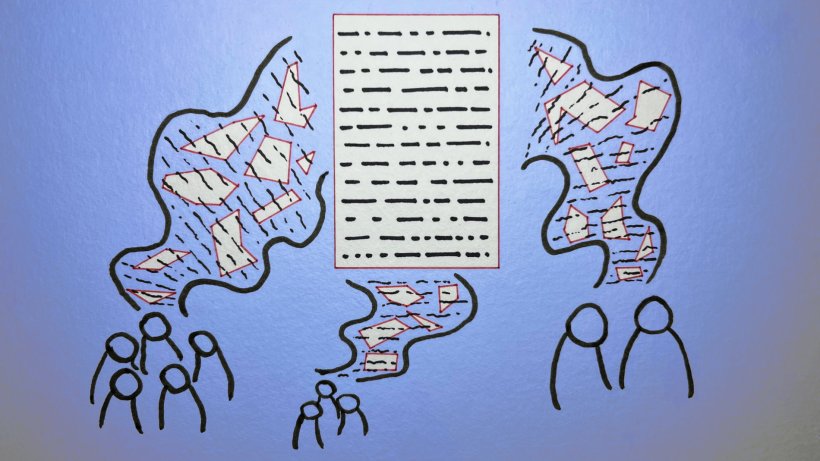
Image source: Yasmine Boudiaf & LOTI / Data Processing as part of a Better Images of AI workshop at Science Gallery London (CC-BY 4.0)
News • LLM exaggerations and overgeneralizations
Generative AI routinely blows up science findings
When summarizing scientific studies, large language models (LLMs) like ChatGPT and DeepSeek produce inaccurate conclusions in up to 73% of cases.
This is according to a new study published in the journal Royal Society Open Science by Uwe Peters (Utrecht University) and Benjamin Chin-Yee (Western University, Canada/University of Cambridge, UK). The researchers tested the most prominent LLMs and analyzed thousands of chatbot-generated science summaries, revealing that most models consistently produced broader conclusions than those in the summarized texts. Surprisingly, prompts for accuracy increased the problem and newer LLMs performed worse than older ones.
Students, researchers, and policymakers may assume that if they ask ChatGPT to avoid inaccuracies, they’ll get a more reliable summary. Our findings prove the opposite
Uwe Peters
The study evaluated how accurately ten leading LLMs, including ChatGPT, DeepSeek, Claude, and LLaMA, summarize abstracts and full-length articles from top science and medical journals (e.g., Nature, Science, and Lancet). Testing LLMs over one year, the researchers collected 4,900 LLM-generated summaries. Six of ten models systematically exaggerated claims found in the original texts often in subtle but impactful ways, for instance, changing cautious, past-tense claims like “The treatment was effective in this study” to a more sweeping, present-tense version like “The treatment is effective.” These changes can mislead readers into believing that findings apply much more broadly than they actually do.
Strikingly, when the models where explicitly prompted to avoid inaccuracies, they were nearly twice as likely to produce overgeneralized conclusions than when given a simple summary request. “This effect is concerning,” Peters said: “Students, researchers, and policymakers may assume that if they ask ChatGPT to avoid inaccuracies, they’ll get a more reliable summary. Our findings prove the opposite.”
Recommended article
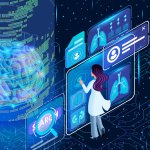
Article • From chatbot to medical assistant
Generative AI: prompt solutions for healthcare?
Anyone who has exchanged a few lines of dialogue with a large language model (LLM), will probably agree that generative AI is an impressive new breed of technology. LLMs show great potential in addressing some of the most urgent challenges in healthcare. At the Medica tradefair, several expert sessions were dedicated to generative AI, its potential medical applications and current caveats.
Peters and Chin-Yee also directly compared chatbot-generated to human-written summaries of the same articles. Unexpectedly, chatbots were nearly five times more likely to produce broad generalizations than their human counterparts. “Worryingly”, said Peters, “newer AI models, like ChatGPT-4o and DeepSeek, performed worse than older ones.”
Why are these exaggerations happening? “Previous studies found that overgeneralizations are common in science writing, so it’s not surprising that models trained on these texts reproduce that pattern”, Chin-Yee noted. Additionally, since human users likely often prefer LLM responses that sound helpful and widely applicable, through interactions, the models may learn to favor fluency and generality over precision, Peters suggested.
The researchers recommend using LLMs such as Claude, which had the highest generalization accuracy, setting chatbots to lower ‘temperature’ (the parameter fixing a chatbot’s ‘creativity’), and using prompts that enforce indirect, past-tense reporting in science summaries. Finally, “If we want AI to support science literacy rather than undermine it,” Peters said, “we need more vigilance and testing of these systems in science communication contexts.”
Source: Utrecht University
14.05.2025