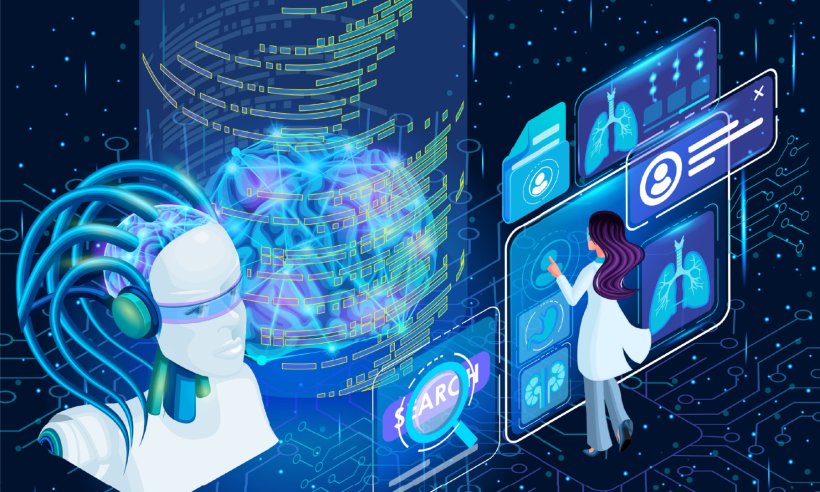
© elizaliv – stock.adobe.com
Article • From chatbot to medical assistant
Generative AI: prompt solutions for healthcare?
Anyone who has exchanged a few lines of dialogue with a large language model (LLM), will probably agree that generative AI is an impressive new breed of technology. Beyond their use as chatbots, LLMs show great potential in addressing some of the most urgent challenges in healthcare. At the Medica tradefair in Düsseldorf, several expert sessions were dedicated to the topic of generative AI, its potential medical applications and current caveats.
Article: Wolfgang Behrends
The above fact that almost everyone has tried their hands at interaction with a chatbot is testament to what some experts believe to be a key benefit of the technology: ‘LLMs are a game changer in that they make generative AI approachable for more people, not just data scientists,’ explained Dr Bertram Weiss during a discussion at the Medica Health IT Forum. ‘This is because you can talk to them using normal language instead of code,’ the Pharma Lead from Berlin-based AI developer Merantix Momentum added.
It is this high level of accessibility that could help hospitals offload a number of basic, but time-consuming tasks, such as appointment scheduling, or patient case documentation. For example, generative AI has shown promising results in transcribing doctor’s notes into structured formats, said co-panellist Dario Antweiler from the Fraunhofer Institute for Intelligent Analysis and Information Systems IAIS. Even though results still need to be checked by a human, ‘this takes away hours of daily work from medical professionals,’ the expert pointed out.
Everyone hallucinates sometimes
Pointing out the need for human validation of the AI-generated content brought about one of the technology’s major issues: results that look plausible at first glance but may turn out to be inaccurate or even completely made up by the algorithm – a phenomenon that has gained notoriety as AI “hallucinations”.
We should establish fair comparisons, to not vilify this powerful technology
Dario Antweiler
Since these fabricated findings cannot always reliably be excluded, safeguards should be established, suggested Antweiler. For example, different AI models could be running independently on the same task to reveal inconsistencies. Also, human operators should always be aware of the possibility of such errors. ‘However, we should not forget that humans also “hallucinate” – we make mistakes, and we tend to be less critical about them than we are about AI hallucinations,’ the expert cautioned. ‘Therefore, we should establish fair comparisons, to not vilify this powerful technology.’
Baked-in bias
Prof Dr Reinhard Heckel pointed to insufficient data as a major cause for underperforming AI models. Rare conditions are by nature underrepresented in most datasets, and variations in image quality may increase existing misinterpretations, the expert from the Technical University of Munich’s School of Computation, Information and Technology said, adding that inclusion of diverse data could mitigate some of these issues. However, some technologies have biases deeply embedded into their functionality, he explained. For example, some imaging modalities are optimized for lighter skin tones, which inherently leads to superior results in these patients. ‘This is a social issue,’ Antweiler said, pointing out the need to establish groups that should be especially protected from this kind of bias.
Of further note were the IT requirements of generative AI, especially regarding computing power. More general models are often considerably more demanding, barring their use for solutions that need to be locally based to ensure data security. In these cases, smaller, more specific AI models should be implemented, the experts agreed.
Need for “AI literacy” among medical professionals
We should not blindly push for implementation when the side-effects are unclear
Bertram Weiss
Despite the current shortcomings of generative AI models, many experts consider their clinical adaptation to be almost inevitable. Heckel noted that US-based companies are especially adept at translating AI models to marketable products, while European developers tend to have more high-end models, but are more cautious in their implementation. In medical settings, an AI may process highly sensitive data, which calls for a thorough analysis of benefits and potential risks, Weiss pointed out. ‘We should not blindly push for implementation when the side-effects are unclear,’ he concluded.
The aforementioned US companies were of course also at the tradefair, to present their generative AI solutions. Notably, Hadas Bitram, Partner General Manager, Health AI at Microsoft Health & Life Sciences, addressed the issue of responsible use of medical AI at the Medica Innovation Forum. She highlighted safeguards in the company’s own products against AI hallucinations and omissions. For example, the LLM gives reference to the sources a given information is based on, to enable validation and provide clinical context. ‘Still, there should always be an accountable medical professional in the loop,’ Bitram said.
The expert also highlighted “AI literacy” as a crucial skill for future clinicians: ‘The work of medical professionals will become increasingly sophisticated,’ she predicted. Assessing and using the right AI tools, interpreting their results and making the right calls based on their input will therefore be essential. AI may not replace doctors, she concluded, but doctors who use AI will replace those who don’t.
13.01.2025