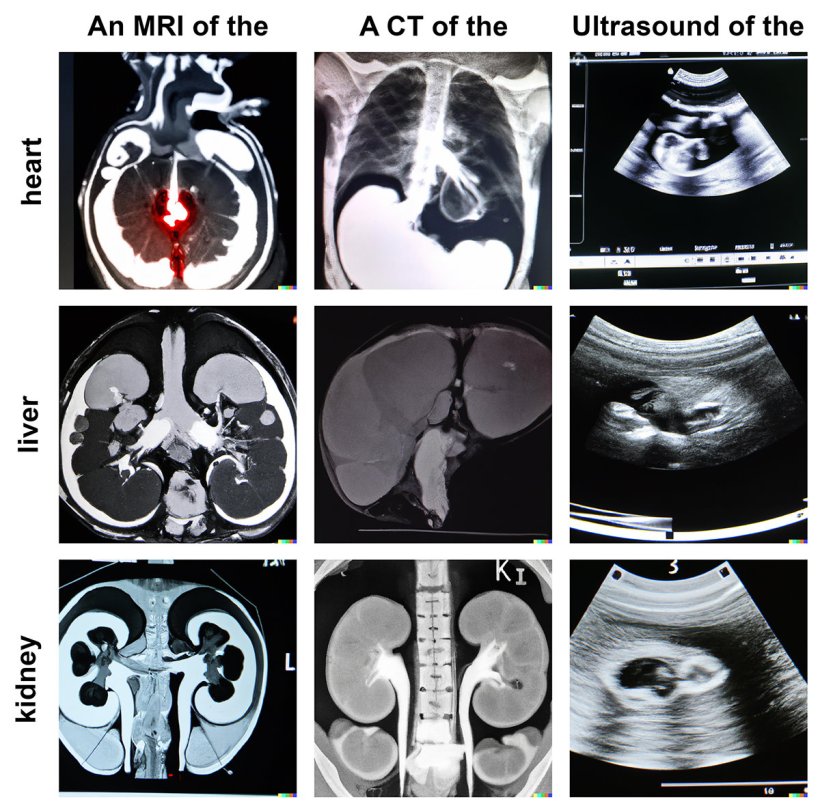
Image source: Adams et al., Journal of Medical Internet Research 2023 (CC BY 4.0)
News • Deep learning text-to-image
Can AI image generator DALL-E 2 advance radiology?
A new paper describes how generative models such as DALL-E 2, a novel deep learning model for text-to-image generation, could represent a promising future tool for image generation, augmentation, and manipulation in health care.
Do generative models have sufficient medical domain knowledge to provide accurate and useful results? Dr. Lisa C Adams and colleagues explore this topic in their latest viewpoint titled "What Does DALL-E 2 Know About Radiology?", published in the Journal of Medical Internet Research.
First introduced by OpenAI in April 2022, DALL-E 2 is an artificial intelligence (AI) tool that has gained popularity for generating novel photorealistic images or artwork based on textual input. DALL-E 2's generative capabilities are powerful, as it has been trained on billions of existing text-image pairs off the internet.
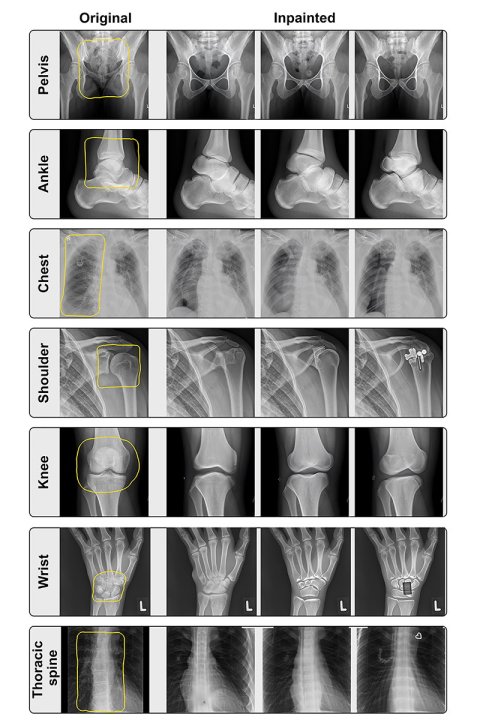
Image source: Adams et al., Journal of Medical Internet Research 2023 (CC BY 4.0)
To understand whether these capabilities can be transferred to the medical domain to create or augment data, researchers from Germany and the United States examined DALL-E 2's radiological knowledge in creating and manipulating X-ray, computed tomography (CT), magnetic resonance imaging (MRI), and ultrasound images.
The study's authors found that DALL-E 2 has learned relevant representations of X-ray images and shows promising potential for text-to-image generation. Specifically, DALL-E 2 was able to create realistic X-ray images based on short text prompts, but it did not perform very well when given specific CT, MRI, or ultrasound image prompts. It was also able to reasonably reconstruct missing aspects within a radiological image. It could do much more—for example, create a complete, full-body radiograph by using only one image of the knee as a starting point. However, DALL-E 2 was limited in its capabilities to generate images with pathological abnormalities.
Synthetic data generated by DALL-E 2 could greatly accelerate the development of new deep learning tools for radiology, as well as address privacy concerns related to data sharing between institutions. The study's authors note that generated images should be subjected to quality control by domain experts to reduce the risk of incorrect information entering a generated data set. They also emphasize the need for further research to fine-tune these models to medical data and incorporate medical terminology to create powerful models for data generation and augmentation in radiology research.
Although DALL-E 2 is not available to the public to fine-tune, other generative models like Stable Diffusion are, which could be adapted to generate a variety of medical images.
Overall, this viewpoint provides a promising outlook for the future of AI image generation in radiology. Further research and development in this area could lead to exciting new tools for radiologists and medical professionals.
Source: JMIR Publications
17.03.2023