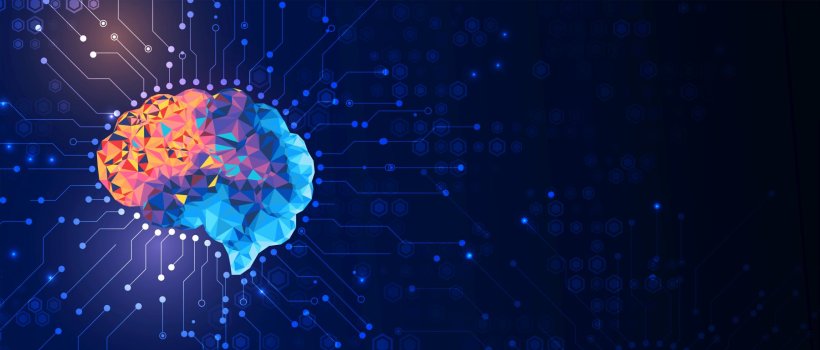
Image source: Adobe Stock/kras99
News • AI in intensive care units
Assistance of AI in the decision-making process of clinicians in intensive care units
Clinicians in an intensive care unit (ICU) need to make complex decisions quickly and precisely, monitoring critically ill or unstable patients around the clock. Researchers from Carnegie Mellon University's Human-Computer Interaction Institute (HCII) collaborated with physicians and researchers from the University of Pittsburgh and University of Pittsburgh Medical Center (UPMC) to determine if artificial intelligence could help in this decision-making process and if clinicians would even trust such assistance.
The team gave 24 ICU physicians access to an AI-based tool designed to help make decisions and found that most incorporated the assistance into some of their decisions. The paper has recently been published in
"It feels like clinicians are excited about the potential for AI to help them, but they might not be familiar with how these AI tools would work. So it's really interesting to bring these systems to them," said Venkatesh Sivaraman, a Ph.D. student in the HCII and member of the research team.
Sivaraman presented the team's paper "Ignore, Trust or Negotiate: Understanding Clinician Acceptance of AI-Based Treatment Recommendations in Health Care" this month at the Association for Computing Machinery's Conference on Human Factors in Computing Systems (CHI 2023) in Hamburg, Germany.
The interactive clinical decision support (CDS) interface
The idea is that maybe we can learn from some of that data so we can try to speed up some of their processes, make their lives a little bit easier and also maybe improve the consistency of care.
Venkatesh Sivaraman
Using the AI Clinician model introduced in Nature by a group of researchers in 2018, the team designed an interactive clinical decision support (CDS) interface - called the AI Clinician Explorer—that provides recommendations for treating sepsis. The model was trained on a data set of more than 18,000 patients who met standard diagnostic criteria for sepsis at some point during their ICU stays. The system enables clinical experts to filter and search for patients in the data set, visualize their disease trajectories, and compare the model predictions to actual treatment decisions delivered at the bedside.
"Clinicians are always entering a lot of data about the patients they see into these computer systems and electronic health records," Sivaraman said. "The idea is that maybe we can learn from some of that data so we can try to speed up some of their processes, make their lives a little bit easier and also maybe improve the consistency of care."
The team put their system to the test via a think-aloud study with 24 clinicians who practice in the ICU and have experience treating sepsis. During the study, participants used a simplified AI Clinician Explorer interface to assess and make treatment decisions for four simulated patient cases.
The results provided insight on ways to improve the AI Clinician Explorer
"We thought the clinicians would either let the AI make the decision entirely or ignore it completely and make their own decision," Sivaraman said.
But the results were not so binary. The team identified four common behaviors among the clinicians: ignore, rely, consider and negotiate. The "ignore" group did not let the AI influence their decision and mostly made their decisions before even looking at the recommendation.
By contrast, the "rely" group consistently accepted at least part of the AI's input in every decision. In the "consider" group, physicians thought about the AI recommendation in every case and then either accepted or rejected it. Most participants, however, fell into the "negotiate" group, which includes practitioners who accepted individual aspects of the recommendations in at least one of their decisions, but not all.
The team was surprised by these results, which also provided insight on ways to improve the AI Clinician Explorer. Clinicians expressed concerns that the AI did not have access to more holistic data, such as the patient's general appearance, and were skeptical when the AI made recommendations contrary to what they were taught.
"When the CDS deviates from what clinicians would normally do or consider to be best practice, there was not a good sense of why," Sivaraman said. "So right now, we're focusing on determining how to provide that data and validate these recommendations, which is a challenging problem that will require machine learning and AI."
Recommended article
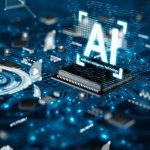
News • Artifical Intelligence
The promise—and pitfalls—of medical AI headed our way
Doctors across all disciplines, with assistance from artificial intelligence, may soon have the ability to quickly consult a patient's entire medical file against the backdrop of all medical health care data and every published piece of medical literature online.
The team's research doesn't attempt to replace or replicate clinician decision-making, but instead hopes to use AI to reveal patterns that may have gone unnoticed in past patient outcomes.
"There are a lot of diseases, like sepsis, that might present very differently for each patient, and the best course of action might be different depending on that," Sivaraman said. "It's impossible for any one human to amass all that knowledge to know how to do things best in every situation. So maybe AI can nudge them in a direction they hadn't considered or help validate what they consider the best course of action."
Sivaraman's collaborators include Adam Perer, an assistant research professor in the HCII; Leigh Bukowski, a senior research manager at the University of Pittsburgh's School of Public Health; Joel Levin, a doctoral candidate in Pitt's Katz Graduate School of Business; and Jeremy Kahn, a physician in UPMC's Department of Critical Care Medicine and associate professor of critical care medicine and health policy in Pitt's School of Medicine and School of Public Health.
Source: Carnegie Mellon University
29.04.2023