Article • The role of chest CT in diagnosis and treatment
UPDATE: Covid-19 and lung infections imaging
RSNA 2020: International experts showcased new studies on chest CT’s role in Covid-19 diagnosis and treatment, and how a smartphone-operated algorithm can help detect TB on plain chest films.
Report: Mélisande Rouger
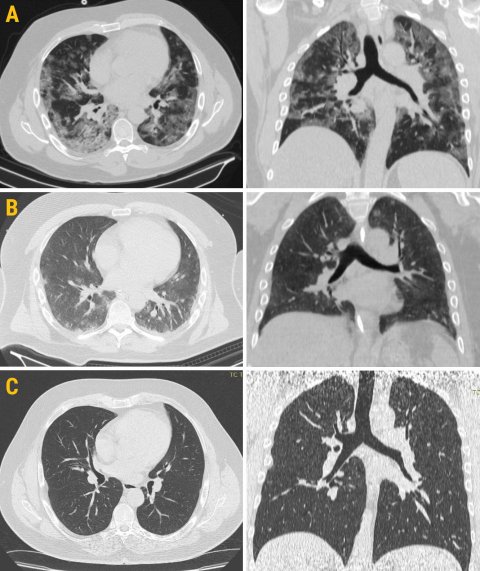
Images courtesy of Marcello Petrini
A staggering volume of work has been produced on the pandemic this year, with an average 367 Covid-19 journal articles published per week, according to Michael Chung, Assistant professor of radiology at Icahn School of Medicine at Mount Sinai, NYC, who quoted a recent study in Nature and Human Behaviour in his keynote on Sunday, the first day of this year’s RSNA gathering.
Radiologists have been actively participating in the effort and collaborated to provide much needed statements and guidelines that were backed by scientific societies and health organisations, such as the Fleischner Society and the WHO. With the boom in Covid-19 imaging research, there is only a natural progression for AI-related projects to enter the landscape, Chung explained. ‘AI has the exciting potential to expand the role of imaging in Covid-19 beyond just diagnosis to even enable patient stratification and to forecast treatment effects,’ he said. But AI has also been explored to better image other diseases that affect the lungs and continue to impact global health, such as tuberculosis (TB), a speaker explained in the session.
Smartphone-operated AI to reduce inequalities in AI opportunities
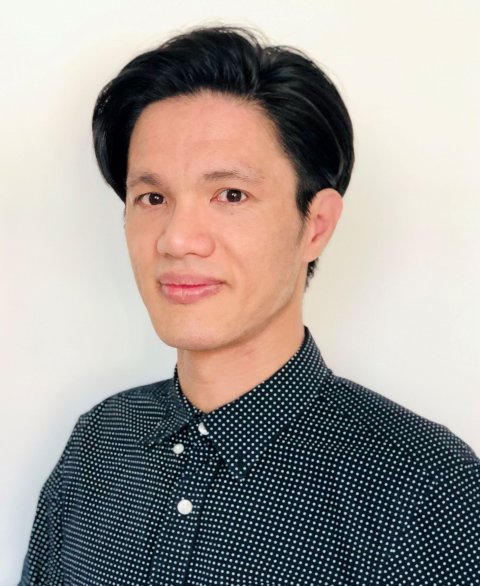
Current advances in medical imaging only apply to rich countries with models that are developed on high resolution images, according to Po-Chih Kuo, assistant professor at Department of Computer Science, National Tsing Hua University in Taiwan. ‘The value of medical AI is unfulfilled if it widens the health disparities between rich and poor countries. We need to extend the opportunities around medical AI to resource-limited settings, ‘ said Kuo as he presented his research on transferring deep learning for TB detection on chest x-ray (CXR) images captured by phone camera.
TB has a poor outcome in low-income countries due to shortage of radiologists, and more than a third of the estimated TB cases are not diagnosed and not reported. In 2019, a study using seven TB datasets to evaluate accuracy of DL model in TB detection achieved more than 0.997 in AUC, suggesting that DL can perfectly distinguish TB in normal patients from their chest x-ray images, Kuo explained. ‘This seemed like a perfect ending to the story, but in some places where we would like to screen TB patients CXR is not digitised. This is the stage where smartphone-operated algorithms for automated reading of chest x-ray can impact,’ he said.
Kuo and colleagues explored the possibility of applying state-of-the-art AI to traditional chest x-ray (CXR) films, by taking photographs on CXRs and interpreting them using smartphones. The result is an algorithm, called TBShoNet, which can be deployed on smartphones to assist healthcare providers in resource-limited areas. In his study, Kuo used publicly available datasets from MIMIC-CXR, Beth Israel Deaconess Medical Centre in Boston, Montgomery Department of Health and Human Services, Maryland, and Shenzhen, the third public hospital in China, for pre training transferring and evaluation. A 121-layer neural network was pre trained on MIMIC-CXR database, containing 215,000 CXR with 14 pulmonary labels that did not include TB cases.
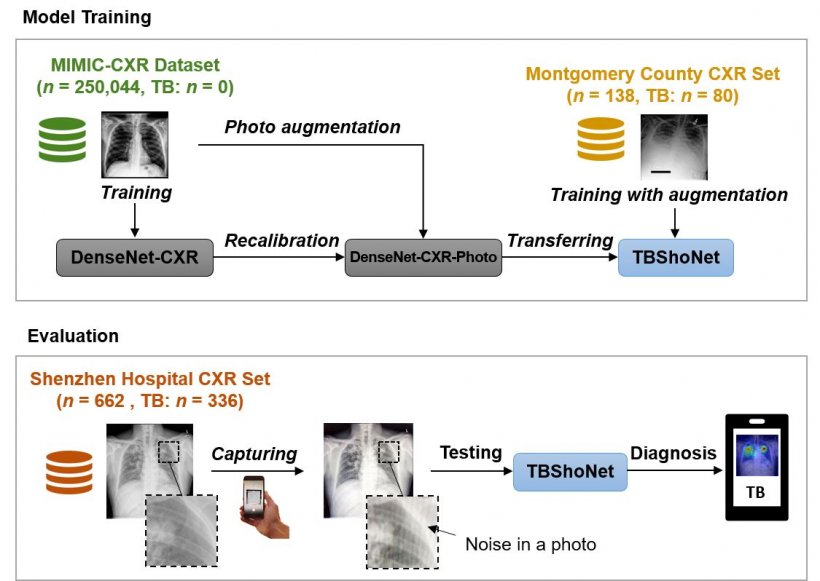
The researchers pre trained the DenseNet by using the dataset, and then recalibrated this model into a photo-specific model by using augmentation functions. This model was transferred into an algorithm called TBShoNet, by training a small dataset including 80 TB cases. Finally, they evaluated the model by taking the real photo from Shenzhen hospital CXR datasets. All photos from CXR were used as training data from TBShoNet and results were evaluated by looking at detection accuracy between TB and normal patients. When testing photos, the TBShoNet demonstrated an AUC of 0.89 for TB detection, and sensitivity and sensibility for classification of 81% and 84%, respectively.
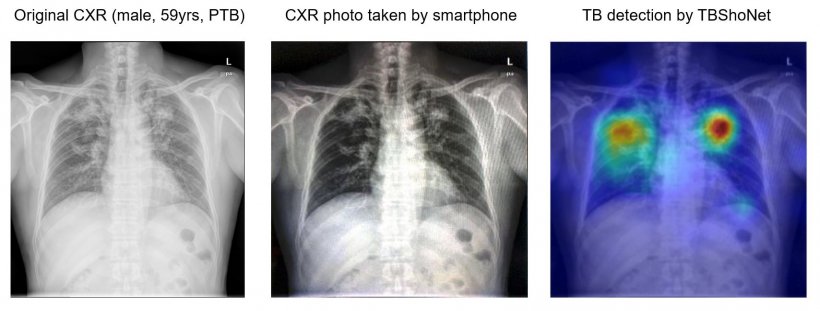
DL algorithm with chest CT can help predict ICU admission
A multinational team of researchers investigated the potential for 3D deep learning (DL) to predict clinical outcomes of patients with Covid-19 based on chest CT, using thousands of images from China, Japan, Italy and the United States. ‘There are many efforts using AI to detect Covid-19, but there are still critical challenges due to data diversity issues,’ said Dr Ziyue Xu, senior scientist with NVIDIA Corporation, a multinational technology company based in Santa Clara, California. The researchers correlated 632 chest CT scans in patients diagnosed with Covid-19 with the clinical outcome. Of the 632 patients, 69 were admitted to the ICU.
An automated whole lung segmentation algorithm was developed and used to localise the whole lung. Based on the local region-of-interest around the lung, a 3-D DL-based algorithm for classification of clinical outcome labels was trained with five-fold cross validation. Classification models achieved overall accuracy of 92%, sensitivity 73%, and specificity 93.9%, despite an imbalanced dataset weighted towards no ICU admission, the authors wrote. The positive predictive value for predicting ICU admission was only 53%, but the negative predictive value was 97%.
Such a model might alert the clinician to the enhanced potential of ICU admission, when combined with other clinical features, Xu explained. ‘Based upon chest CT alone, AI-based deep learning algorithms can reasonably predict clinical outcomes, such as ICU admission, in patients with Covid-19 who underwent CT and PCR on the day of admission. The model is feasible with reasonable accuracy and specificity of prediction,’ he confirmed. However, the current study should be interpreted with caution, and is preliminary and limited in its ‘unbalanced data, small number of positive cases, single site, and lack of inclusion of standard clinical metrics,’ he added.
The importance of prevalence in chest CT predictive value
Positive predictive value (PPV) and negative predictive value (NPV) of chest CT are strongly influenced by disease prevalence, a team of Italian researchers from Guglielmo da Saliceto Hospital Piacenza has just shown. The researchers evaluated the diagnostic performance of CT for severe Covid-19 pneumonia in two different epidemic phases at their hospital, where some of the first European patients with Covid were admitted last February. The team analysed admission chest CT scans of patients with suspected Covid-19 pneumonia and who also underwent reverse transcriptase-polymerase chain reaction (RT-PCR) of nasal-pharyngeal swab.
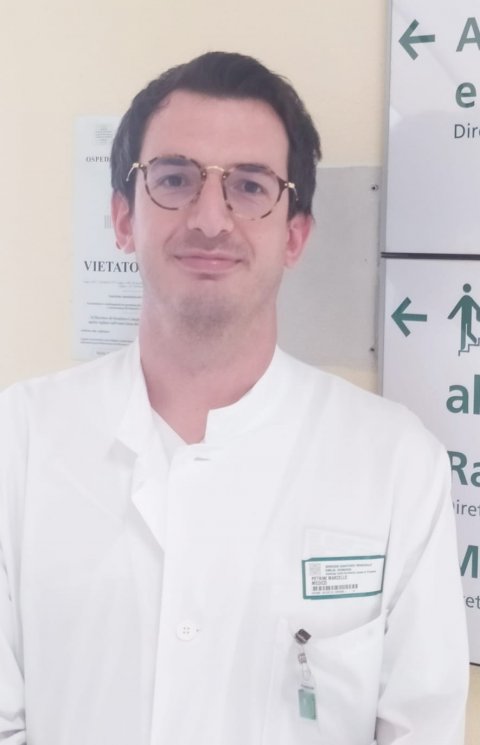
The first set of images was acquired in 198 patients admitted in the outbreak period between 21 February and 7 March and who had a positive swab ratio of 89%. The second group included 146 patients who were admitted in 6-13 April, after 28 days of lockdown, so in a period of reduced prevalence (positive swab ratio of 31%). RT-PCR result was used as reference standard to calculate CT sensitivity, specificity, positive PPV and NPV in the two periods.
While results confirm CT’s high sensitivity and low specificity for Covid-19 pneumonia in both groups, as suggested by earlier literature, the Italian researchers found that PPV and NPV differ depending on disease prevalence. ‘Given a negative CT, the likelihood of having Covid-19 pneumonia is 42% (NPV:58%) during the high prevalence phase, while it is 4% (NPV: 96%) in the low prevalence period,’ said Marcello Petrini, a radiologist involved in the study.
Although all retrospective studies have a low level of evidence, this new study shows that radiologists must look beyond sensitivity and specificity in their management of patients with Covid pneumonia. ‘PPV and NPV should drive clinical management of patients with suspected Covid-19 pneumonia,’ Petrini concluded.
Profiles:
Dr. Po-Chih Kuo (郭柏志) is an assistant professor at the Department of Computer Science, National Tsing Hua University (NTHU), Taiwan. He received his PhD and BSc. degree from the Institute of Computer Science and Engineering at National Chiao Tung University (NCTU) and Computer Science and Information Engineering at National Cheng Kung University (NCKU), respectively. He was a postdoctoral research fellow in the functional neuroimaging lab at the Institute of Statistical Science, Academia Sinica, Taipei, Taiwan, and a postdoctoral fellow in the Laboratory for Computational Physiology at Institute for Medical Engineering & Science, MIT. Po-Chih Kuo has been involved in research projects with the Swartz Centre for Computational Neuroscience, University of California, San Diego, where he developed visual imagery BCI, and at the Institute of Statistical Mathematics, Tokyo, Japan, where he worked on statistical thresholding method in non-stationary fMRI data. Also, at the Institute of Neuroscience and Medicine in Research Centre Jülich, he applied machine learning methods on neurotransmitter receptor images for brain segmentation.
Ziyue Xu joined NVIDIA as a Senior Scientist in 2018, after being a Staff Scientist and Lab Manager at National Institutes of Health. His research focus is on image analysis and computer vision, with applications in biomedical and clinical imaging using shape modeling, graph methods and machine learning. He has worked on medical AI for several years along with fellow researchers and clinicians. Ziyue gained a BSc at Tsinghua University in 2006, and MSc/PhD from the University of Iowa in 2009/2012. He is an Associate Editor for the journals, Computerised Medical Imaging and Graphics (CMIG), IEEE Transactions on Medical Imaging (TMI), the Journal of Biomedical and Health Informatics (JBHI), and Computers in Biology and Medicine (CBM).
Marcello Petrini is a radiologist at Guglielmo da Saliceto Hospital in Piacenza, Italy. He completed his studies at University of Milan and had a fellowship in cardiac imaging in Vienna, Austria. His European Diploma in Radiology was gained in 2017. His subspecialties are cardiac and emergency radiology. The Covid-19 pandemic that spread in Europe began near Piacenza Guglielmo da Saliceto Hospital. Together with the hospital director, Dr Michieletti, and Dr Colombi, a chest radiologist, Petrini tried to study Covid-19 imaging, publishing articles in Radiology about CT predicting outcome, and in European Radiology on CT and ultrasound comparisons, plus in Emergency Radiology about CT qualitative and quantitative chest CT parameters as predictors of specific mortality.
03.12.2020