Integrating speech recognition with a RIS
A report turnaround time decrease of 50% leads doctors to plan for 100% usage of the dictation system.
Interview: Armin Scheuer of HealthTech Wire.
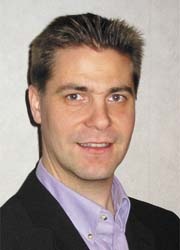
The Helsinki University Central Hospital, in Finland, piloted speech recognition at Toolo Hospital’s radiology department to evaluate its effects on report generation. The Technology Manager, Tomi Kauppinen PhD, presented the pilot’s first results at the 2005 European Radiology Congress (ECR). These indicated a 50% decrease in report turnaround time. At the 2006 congress, he followed up on the study, explaining how the pilot hospital plans to increase the number of reports processed through speech recognition from 70% to almost 100%.
‘We are a trauma centre, so emergency reports are needed fast, or instantly, Tomi Kauppinen explained. ‘We used to dictate on cassettes, or handwrite reports. But, in modern healthcare this process is not acceptable, as it is error-prone and time consuming for radiologists. Although several European and US studies have suggested that radiologists who dictate, edit and validate their reports themselves work less time-efficiently, our experience has proved the contrary. In the beginning, eliminating deferred transcription slowed radiologists down, but after their training, reporting turn-around decreased, which for us was a major motivation to introduce speech recognition (SR). Several steps in the process, such as secretarial queries, manual typing, or phone calls from those awaiting reports, have been eliminated, so overall workflow and productivity have improved, rather than declined. Achieving a 50% decrease in report turn-around was easier than we thought. Corrections are also minimal, because we trained the system and are constantly updating recognition vocabularies and adding unknown words - a key feature in making front-end speech recognition successful. A dedicated administrative person manages these updates and adaptations.
When introducing speech recognition, what other aspects need consideration?
Ideally, SR should become a natural part of the RIS, so I would roll out the RIS and SR jointly, so that users need to adapt to only one new system. I’d also recommend demanding that RIS providers deliver a SR-based solution. Then, when you integrate the RIS with PACS, you get a truly homogeneous infrastructure that binds all radiology applications in one integrated solution.
You aim for 100% processing of radiology reports using speech recognition?
As a teaching hospital we will always create some reports traditionally, for training purposes. Currently, 70% of our reports are created with SR; we could raise this to almost 100%. Optimising integration with the RIS and RIS user interface is one aspect; another is the availability of SR at every workstation - ideally you should be able to use it from every RIS workstation, which is why I recommend rolling out RIS and SR jointly. The third step is to optimise RIS/PACS integration, because an integrated SR system depends on seamless communication between both leading applications. This means that radiologists could choose a patient from a list in the RIS, and the PACS automatically delivers corresponding images. It’s a quality and security step, ensuring images and reports correspond with the correct patient. It is also the basis for starting to use the SR application in the RIS. In fact, it further adds to time-saving in the overall reporting workflow.
How did you convince your radiologists to switch from cassettes to speech recognition?
User acceptance is a critical point and we underestimated it. People fear change and good, clear information in advance eliminates negative expectations. The integrated application is very user-friendly - however, investing time to explain it to users, supporting them with training their voice profiles, and showing them how straightforward the system is, will lead to fast user acceptance.
On average, training took 1-2 hours per user. Given the importance of PACS/RIS/SR to the department, a senior radiologist on the core team trains new users himself. We also implemented a help desk to give immediate support to users with problems, but it can’t really complain about much work. In fact, we had only a few technical difficulties with the SR system.
At the ECR you mentioned that reporting quality improved.
Yes. First, because radiologists know their complex vocabulary, so immediately check whether a word was recognised correctly. A transcriber might make spelling errors, but radiologists no longer have to spell out difficult terms for them. Secondly, radiologists now dictate in a more structured and organised format, because they see their report directly on screen. This also adds to a final report’s clarity and quality.
So why don’t all radiology departments use SR?
Many RIS vendors don’t offer an integrated solution, which is why I recommend that my colleagues avoid SR-isolated implementations on individual workstations; the experience will be disappointing. Instead, they should pressure their RIS providers to integrate SR in their system and help them to implement a structured reporting workflow that takes into account all involved applications - PACS, RIS and speech recognition.
01.07.2006