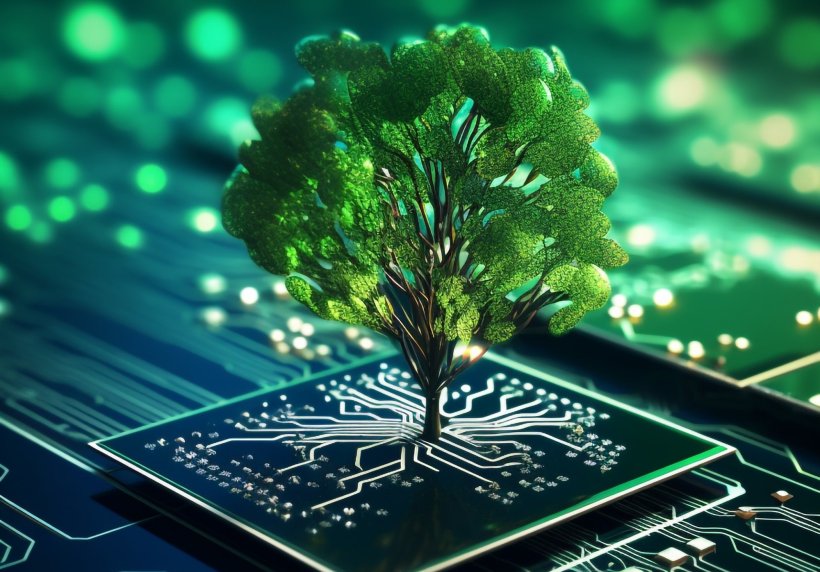
Image source: Adobe Stock/Starmarpro (generated with AI)
Article • Experts explore impact of technology
AI in radiology: helper or bane of society and the environment?
The climate crisis and AI – arguably two of the most hotly-debated and relevant topics of our time – share an intricate relationship: While computation of complex AI routines commands an immense carbon footprint, it is these algorithms that might be the very key to mitigate the effects of global warming. In a dedicated session at ECR 2023, radiologists explored the societal and environmental impact of the technology in healthcare.
Report: Mélisande Rouger
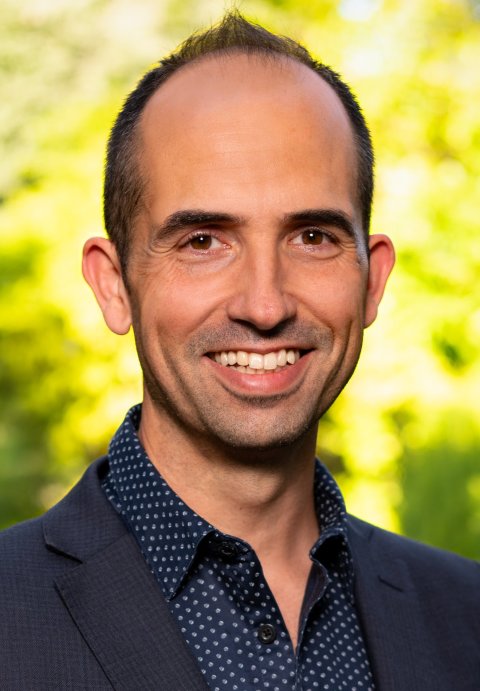
Angel Alberich-Bayarri, CEO of Valencia-based AI company Quibim, looked at the technology’s carbon footprint and the strategies to make AI sustainable in the opening talk. ‘AI is the new electricity, and it will continue to change the world,’ he told the audience. ‘The power for positive change that AI brings holds the possibility for negative impacts on society.’
This impact is felt, among others, in the massive amount of CO2 that is being generated when developing a new algorithm. ‘Training a single deep learning, natural language processing algorithm can take approximately 600,000 pounds of carbon emissions,’ the expert explained. ‘It’s the same amount of CO2 produced by five cars over their lifetimes.’ For example, training Google AlphaGo Zero, a tool developed to solve the Go game, generated 96 tons of CO2 over 40 days – the same amount as 1,000 hours of air travel.
To date, sustainability has barely been explored in AI ethics, a field that studies ethical and societal issues facing developers, producers, consumers, citizens, policy makers and civil society organizations. Alberich-Bayarri expressed his conviction that times are calling for a change and sustainability must now come into the limelight. ‘Sustainable AI is in its infancy: it’s underdeveloped, under researched and underfunded, but we’re starting to explore it. It’s time to analyse the environmental impact of AI. We can’t neglect the carbon footprint that we are creating when training a model.’
Efforts to direct AI uses towards good purposes are increasing, but there are no real business tools available to measure AI’s carbon footprint yet. ‘We’re starting to get concerned about the impact of AI on our environment,’ the Quibim CEO explained the inherent contradiction. While AI is being proposed to power up society, its development and use make society unsustainable at the same time. Deep learning models are posing both financial and environmental costs – from hardware, electricity and cloud computing time to the carbon footprint generated when fuelling modern tensor processing hardware. ‘The energy required to do so is considerable,’ he said.
Tracking, shrinking, specializing: Strategies to reduce AI’s carbon footprint
The goal is to shrink down the size of the models and use fewer computing cycles to decrease financial and environmental costs of building and deploying AI
Angel Alberich-Bayarri
There are already a few tools that AI developers can use to calculate their carbon emissions – for example the Machine Learning Emissions Calculator, to estimate the carbon footprint of GPU computing, and the Carbontracker, an experiment impact tool for real time assessment of energy consumption and carbon emissions. ‘This carbon tracking is being increasingly asked in grant applications or proposals in hospitals to measure impact on the environment. I encourage all of us to track our CO2 emissions.’ There are three main aspects to consider to foster sustainable AI. The first consists in designing smaller models when implementing AI. Several research initiatives are exploring how to train models faster and more efficiently, relying on techniques like pruning, compression, distillation and quantization. ‘The goal is to shrink down the size of the models and use fewer computing cycles to decrease financial and environmental costs of building and deploying AI,’ Alberich-Bayarri said.
The second aspect is to alternate deployment strategies, including the use of specialized hardware like application-specific integrated circuits (ASICs); prevention of idle consumption and sub-optimal computational processing distribution; and optimizing the use of existing hardware like general-purpose CPUs. Last but not least, the AI community must work to improve carbon awareness, the expert appealed. ‘We have to partner with cloud providers who are aware about the issue. Some data processing centres are using immersion-based cooling for different devices, grid interactive UPS batteries or hydrogen batteries instead of diesel generators as a backup for main data processing centres.’
Not in a rush? Keep your data cool
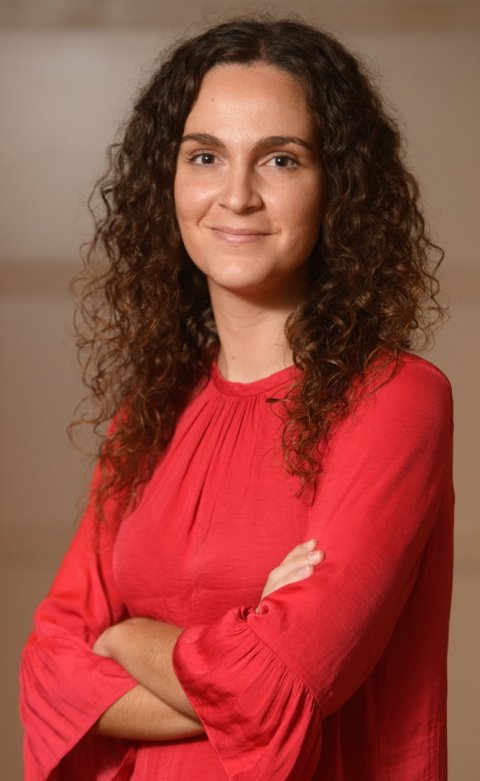
AI developers must also consider where they store the files depending on how quickly they need to be retrieved, he urged. ‘Amazon, for instance, offers different portfolios: we can have a very warm storage with immediate access to data, but if we don’t need to use the data right away, we can store it in an iceberg instead and have the data in hours instead of seconds. That’s another way to reduce our environmental impact.’
Ana Jimenez-Pastor, Vice President of AI at Quibim, then focused on how AI can help mitigate the lack of radiologists in low- to middle-income countries. In these regions, she argued, the global shortage of radiologists is felt more acutely. For example, in Tanzania, roughly 60 radiologists have to tend to a 25 million population, or Kenya, where here are only three fellowship-trained breast radiologists to scan over four million women who need annual mammography. ‘AI could have a great impact in these countries with few to no radiologists available, by automating image interpretation and potentially enabling a few radiologists to manage the workload of hundreds,’ she suggested.
Profiles:
Ángel Alberich-Bayarri is a Telecommunications Engineer with specialisation in electronics by the Technical University of Valencia and PhD in Biomedical Engineering for his research on the application of advanced image processing techniques to magnetic resonance imaging. Founder and CEO of Quibim (Quantitative Imaging Biomarkers in Medicine), a company dedicated to the advanced analysis of medical images using artificial intelligence. He is the author of more than 90 scientific articles in prestigious international journals and inventor of 5+ patents. He is also the author of more than 100 communications to international congresses, editor of international books and author of 20+ book chapters. He has participated in a high number of international research projects and clinical trials. He is an active member of several scientific societies, among which stands out his participation as a member of the Board of Directors of the European Society of Medical Imaging Informatics (EUSOMII).
Ana Jimenez-Pastor is a Telecommunications and Biomedical Engineer from the Polytechnic University of Valencia (Spain). She currently works as VP of AI at Quibim, leading the coordination and development of new and innovative AI solutions applied to the field of radiomics together with MLOPs pipelines to ensure data governance and AI models reproducibility. Ana has dedicated the last seven years to the research, development and integration in clinical practice of solutions that combine image analysis, quantification and AI. Ana has participated and coordinated different European H2020 projects focused on the development of European infrastructures for the study of cancer using AI and image analysis. Author of more than ten publications and book chapters, she has presented her research in different national and international congresses.
15.01.2024