News • Neural network-based assessment
AI calculates biological heart age, cardiovascular risk, from ECG data
While everybody’s heart has an absolute chonological age (as old as that person is), hearts also have a theoretical ‘biological’ age that is based on how the heart functions.
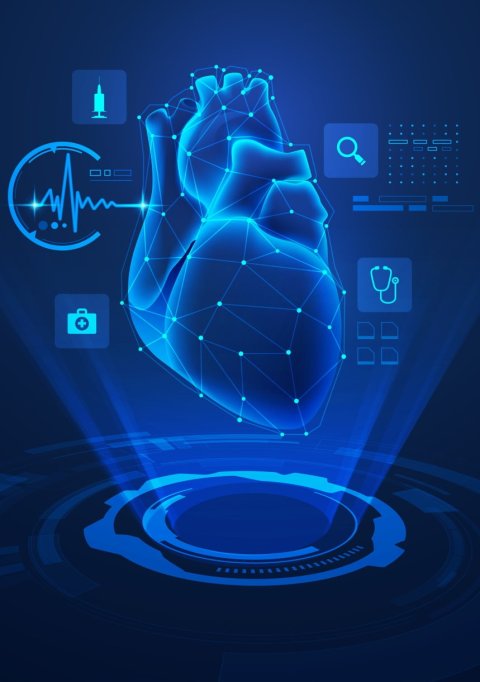
© Jackie Niam – stock.adobe.com
So someone who is 50 but has poor heart health could have a biological heart age of 60, while someone of 50 with optimal heart health could have a biological heart age of 40.
Researchers presenting a new study at EHRA 2025, a scientific congress of the European Society of Cardiology (ESC), demonstrated that by using artificial intelligence (AI) to analyse standard 12-lead electrocardiograph (ECG) data taken from almost half a million cases, they were able to create an algorithm to predict the biological age of the heart. This algorithm could be used to identify those most at risk of cardiovascular events and mortality. “Our research showed that when the biological age of the heart exceeded its chronological age by seven years, the risk of all-cause mortality and major adverse cardiovascular events increased sharply,” explains Associate Professor Yong-Soo Baek, Inha University Hospital, in South Korea. “Conversely, if the algorithm estimated the biological heart as seven years younger than the chronological age, that reduced the risk of death and major adverse cardiovascular events.”
The integration of artificial intelligence (AI) into clinical diagnostics presents novel opportunities for enhancing predictive accuracy in cardiology. “Using AI to develop algorithms in this way introduces a potential paradigm shift in cardiovascular risk assessment,” says Associate Professor Baek.
Recommended article
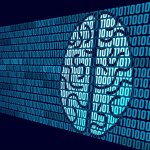
Article • Technology overview
Artificial intelligence (AI) in healthcare
With the help of artificial intelligence, computers are to simulate human thought processes. Machine learning is intended to support almost all medical specialties. But what is going on inside an AI algorithm, what are its decisions based on? Can you even entrust a medical diagnosis to a machine? Clarifying these questions remains a central aspect of AI research and development.
Their study evaluated the prognostic capabilities of a deep learning based algorithm that calculates biological ECG heart age (AI ECG-heart age) from 12-lead ECGs, comparing its predictive power against traditional chronological age (CA) for mortality and cardiovascular outcomes.
This approach will enhance the robustness and applicability of AI ECG in clinical assessments of cardiac function and health
Yong-Soo Baek
A deep neural network was developed and trained on a substantial dataset of 425,051 12-lead ECGs collected over fifteen years, with subsequent validation and testing on an independent cohort of 97,058 ECGs. Comparative analyses were conducted among age and sex-matched patients differentiated by ejection fraction (EF).
In statistical models, an AI ECG-heart age exceeding the heart’s chronical age by seven years was associated with an increased the risk of all-cause mortality by 62% and of MACE by 92%. In contrast, an AI ECG heart age that was seven years younger than its chronological age reduced the risk of all-cause mortality by 14% and MACE by 27%. Additionally, subjects with reduced ejection fraction consistently exhibited increased AI ECG heart ages along with prolonged QRS durations (the time taken for the heart’s electical signal to travel through the ventricles, causing contraction) and corrected QT intervals (the total time needed for the heart’s electrical system to complete one cycle of contraction and relaxation).
The authors explain that the significance of the observed correlation between reduced ejection fraction and increased AI ECG heart ages, alongside prolonged QRS durations and corrected QT intervals, suggests that AI ECG heart age effectively reflects various cardiac depolarisation and repolarisation processes. These indicators of electrical remodelling within the heart may signify underlying cardiac health conditions and their association with ejection fraction (EF). However, Associate Professor Baek explains: “It is crucial to obtain a statistically sufficient sample size in future studies to substantiate these findings further. This approach will enhance the robustness and applicability of AI ECG in clinical assessments of cardiac function and health.” He concludes: “Biological heart age estimated by artificial intelligence from 12-lead electrocardiograms is strongly associated with increased mortality and cardiovascular events, underscoring its utility in enhancing early detection and preventive strategies in cardiovascular healthcare. This study confirms the transformative potential of AI in refining clinical assessments and improving patient outcomes.”
Source: European Society of Cardiology
01.04.2025