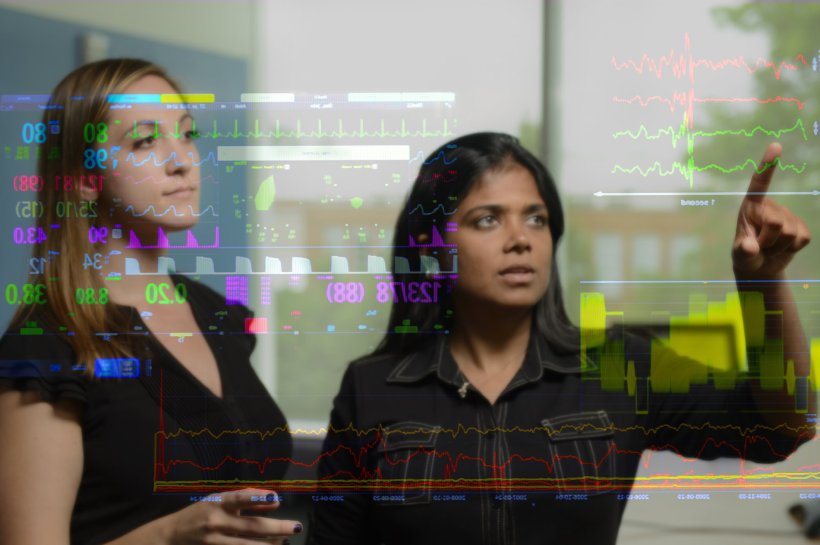
© John Hopkins University
Article • Targeted Real-time Early Warning System for hospitals
Early detection of sepsis with the help of AI
Sepsis, a life-threatening, systemic, toxic bodily reaction to infection, is often difficult to detect in its early stages. Its symptoms, including fever, shortness of breath, rapid heart rate, and confusion, are associated with many medical conditions of hospitalized patients. But if not treated rapidly, a patient may die. The Targeted Real-time Early Warning System (TREWS) for sepsis detection is proving to be a highly effective, lifesaving high-precision bedside early warning system.
Report: Cynthia E. Keen
In Europe, North America, and Australia, the average 30-day rate of sepsis shock mortality is 34.7% and sepsis mortality 24.4%, according to a 2020 meta-analysis of 170 studies published in Critical Care. Automated artificial intelligence (AI) sepsis detection systems in use and in development confirm that alerts at the first indications of sepsis do save lives. But many are criticized for lack of sensitivity and generating too many inaccurate alerts, to the point where they are ignored. TREWS is based on its use for two years monitoring 590,736 inpatients at Johns Hopkins Hospital in Baltimore, Sibley Memorial Hospital in Washington, D.C., and three affiliated area community hospitals. This early warning system has reduced mortality of sepsis patients by nearly 20%.
Patients who had an evaluation and confirmation of sepsis within 3 hours of a TREWS sepsis alert received antibiotics a median time of 1.85 hours earlier than sepsis patients whose conditions were not reviewed in as timely a manner. The “rapid treatment” patient group had a lower mortality rate by 19% and improved sequential organ failure assessment (SOFA) score.
These positive findings, which were published in Nature Medicine, were even more pronounced for sicker, high-risk patients who received rapid antibiotic treatment. In the most severe cases, where an hour delay in diagnosis can mean the difference between life and death, TREWS detected sepsis an average of nearly six hours earlier than when patients were first detected and treated under the current standard of care.
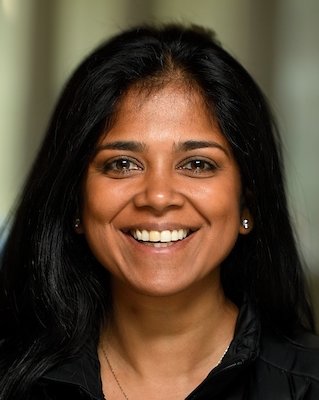
© John Hopkins University
Suchi Saria, PhD, director of the Machine Learning and Healthcare Lab at Johns Hopkins, who led this work, explains that TREWS automatically and continuously monitors disparate clinical data, including vital signs, laboratory data, medication history, procedure and clinical history, and physician notes. It generates a continuous real-time “sepsis score” that can trigger an alert to healthcare staff. Clinical caregivers can analyse why the TREWS alert was generated, accept or dismiss it, and initiate timely treatment on patients confirmed to be septic.
During the study time period, caregivers evaluated 89% of all alerts, 53% within 60 minutes. The researchers attribute this high rate of utilization in part to the fact that the system was tuned to achieve the highest possible performance without generating excessive inaccurate alert warnings. It became trusted by clinical staff.
‘Our results showing high physician adoption and associated mortality and morbidity reductions are a milestone for the field of AI,’ comments Saria. ‘They are the culmination of nearly a decade of significant technological investment, deep collaboration, the development of novel techniques, and rigorous evaluation. Further, what’s most exciting here is that this approach is applicable not just to sepsis but to many other critical complications.’
In an accompanying commentary, Harvard Medical School Professor David W. Bates, MD, an internationally renowned expert in patient safety and use of IT to improve patient care, and Ania Syrowatka MD, PhD, a postdoctoral research fellow specializing in AI applications in medicine, laud the studies as “pivotal” in showing that sepsis detection systems can work well at multiple sites and with a near 90% clinical adoption rate. They caution about the challenges facing widespread adoption of such alert systems. They site the difficulties of implementation across diverse health systems, the expense and difficulty of hospitals adopting multiple AI technologies, and limitations of some EHR systems that do not allow real-time queries of patient EMRs or the capability to route an alert -- such as a sepsis notification – to the most appropriate clinical provider to respond.
But they are positive as well, writing, ‘Overall, these technologies have great potential to improve care and rapidly identify patients who are deteriorating, especially those with sepsis – which could help prevent deaths and reduce costs for large number of patients.’
Profile:
Suchi Saria, PhD, holds the John C. Malone Endowed Chair with faculty appointments within the schools of engineering, public health, and medicine at Johns Hopkins. She also directs the Machine Learning and Healthcare Lab and is the founding research director of the university’s Malone Center for Engineering in Healthcare. Dr Saria is also founder and CEO of Bayesian Health, an AI-based Intelligent Care augmentation platform developer of clinical decision support and detection software designed to increase the capacity of clinicians to visualize and assess risk.
14.11.2022