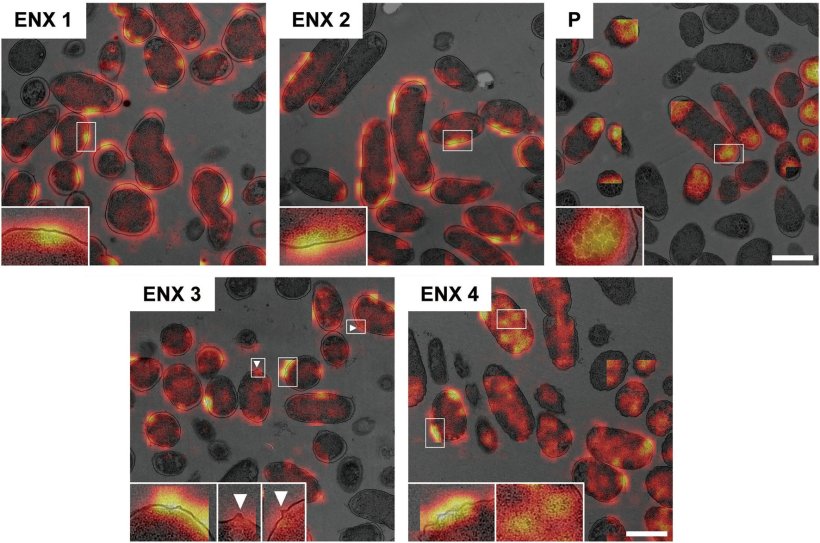
Image source: Hayashi-Nishino et al., Frontiers in Microbiology 2022 (CC BY 4.0)
News • Deep learning approach
'Curvy' bacteria as telltale sign of drug resistance?
Researchers from Osaka University find that drug-resistant bacteria can be distinguished from non-resistant bacteria based on structural changes evident in electron microscope images with high accuracy using deep learning.
The researchers found that bacteria that have developed resistance to antibiotics flaunt their new power in the form of easily detectable shape changes. In a study recently published in Frontiers in Microbiology, the team revealed that machine learning analysis of microscopy images can be used to identify bacteria that are resistant to antibiotics.
Drug resistance is a growing problem worldwide, particularly with the development of multidrug-resistant bacterial strains that can be difficult to control because of the lack of alternative treatment options. Compounding the problem is the fact that testing bacteria for drug resistance can be time-consuming and error-prone, requiring laboratory-based testing and qualitative interpretation. “Typically, bacterial drug resistance is investigated in the laboratory by looking at genetic changes that occur in resistant strains,” says Mitsuko Hayashi-Nishino, lead author of the study. “But drug resistance can involve many such changes, and it can be challenging to determine which ones are actually causing the resistance, so we wanted to see if another approach could be more informative.”
Our findings suggest that bacteria change their morphology when acquiring drug resistance, and that these changes can be reliably detected by a machine-learning algorithm
Mitsuko Hayashi-Nishino
The researchers chose to look at alterations in bacterial shape to determine if these changes could predict or reflect drug resistance. To do this, they took close-up images of drug-sensitive and drug-resistant bacteria using transmission electron microscopy and then applied deep learning to the images to identify features that correlated closely with drug resistance. “The results were very clear,” says lead author Kota Aoki. “We found that the bacteria that were resistant to an antibiotic called enoxacin exhibited differences in cell shape, outer membrane structure, periplasmic space, and granule content and location compared with the drug-sensitive strain,” explains senior author Kunihiko Nishino. In fact, the changes in membrane structure were also strongly associated with mutations in the gene lpp, which encodes a major structural component of the outer membrane. “Our findings suggest that bacteria change their morphology when acquiring drug resistance, and that these changes can be reliably detected by a machine-learning algorithm,” says Hayashi-Nishino.
The study findings taken together point to the success of the research team’s method in visualizing the structural features of drug-resistant bacteria. This new approach is expected to lead to the development of technology that can automatically predict drug resistance based on changes in bacterial shape, without the need for drug-based screening.
Source: Osaka University
19.03.2022