Disambiguation
A new system might help to analyse unstructured clinical documentation, such as lab/pathology results, thus tapping a wealth of hidden information.
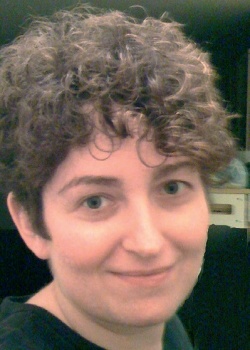
Electronic patient records (EPRs) may harbour a host of clinically useful data, which could bring benefits from the analysis of correlations between symptoms, treatments, and outcomes. The shortlisting of candidates for enrolment in clinical trials would be another potential option.
However, a major obstacle to the extraction of this valuable information from freeform notes has been word-sense disambiguation. The identification of intended meanings in words may now have become possible, computer scientists explained at the 2012 American Medical Informatics Association’s (AMIA) annual symposium in Chicago.
The work on the new disambiguating of words system described was launched at the MIT Computer Science and Artificial Intelligence laboratory (CSAIl) by Dr Anna Rumshisky and Master’s student Rachel Chasin, in the Clinical Decision Making Group headed by Prof. Peter Szolovits. At the time, Dr Rumshisky was a postdoctoral associate; today, she is an Assistant Professor at the Department of Computer Science, University of Massachusetts/lowell, USA.
Asked about the current situation regarding EPRs and options to extract information – particularly lab and pathology results – for research and routine, Dr Rumshisky summed up: ‘Compared to the general domain text, information extraction from unstructured clinical data has been lagging behind, and the main obstacle has been the absence of annotated data for training supervised machine learning systems. This situation is partly due to privacy restrictions on the clinical text.
‘However, over the past six years several large de-identified sets of clinical records have been annotated for different linguistic information, and several community-wide information extraction challenges have pushed the state of the art forward.
These have been organised under the aegis of the Informatics for Integrating Biology and Bedside (i2b2) project and led in large part by the Clinical Decision Making group, with the lead organiser Dr Ozlem Uzuner, a research affiliate and former postdoctoral fellow in the Clinical Decision Making group, currently an assistant professor at SUNY Albany.
Blocked structuring/standardisation and potential approaches
‘The annotated data covers document- level diagnosis extraction, text-level annotations of clinical problems, tests, and treatments/ interventions, as well as relations between them, extraction of medications, and dosages, and a few other information extraction tasks. last year, we ran a challenge on extraction of temporal relations between clinically relevant events, and another annotation effort is under way this year. Importantly, we make the annotated data available to the community for training and testing the automated systems.’
‘Information extraction from clinical text is challenging for a number of reasons. Typically, clinical text is written for experts by experts, and uses shorthand characterised by highly non-standard syntax, which is, at the same time, brimming with abbreviations and acronyms not well documented in any knowledge resources. The most prominent semantic resource used for clinical text processing is the Unified Medical language System (UMlS), which has not been designed with text processing in mind, and therefore is often not directly useful.
‘As far as disambiguation of undocumented acronyms is concerned, for example, creating annotated data would entail (1) devising a sense inventory and (2) annotating a corpus of text for every single ambiguous word. Since this is too labour intensive and therefore expensive, our goal has been to develop unsupervised learning methods to accomplish the same tasks.’
Solution paths
‘We tried several disambiguation methods that do not involve supervised machine learning. The first set of methods used the UMlS to attempt to disambiguate words. For the reasons mentioned (UMlS is not structured appropriately as a linguistic resource) the results were not very impressive. We then decided to adopt a modification of an unsupervised bottom-up probabilistic graphical modelling technique, called topic modelling, which has been used with some success in the general domain.
‘Topic models – in particular, we used latent Dirichlet Allocation and Hierarchical Dirichlet Process – assume that each document was generated from a succession of topics the writer meant to discuss. In turn, each topic generates a set of terms. Topic modelling uses correlations among terms as they are used in a large corpus to suggest what might be the likely succession of topics, and learns probabilities for both topics and the terms they generate. This probabilistic model then permits identification of common topics in this domain, and subsequent recognition of discussions of that topic in future documents.
‘The twist that we’ve adopted from the general domain is to treat each occurrence of the target ambiguous word as a separate document, and to associate the induced hidden topics with the senses of the ambiguous word, which in turn will be associated with the linguistic features characterising each example.
‘Tested on 50 annotated targets, our best results are 85.6% accuracy on targets with two senses, and 66.3% accuracy over all targets combined.
‘We are currently developing a generalised model that integrates more sophisticated linguistic features characterising each ambiguous example, specifically, context features based on syntactic parses and UMlS mapping of context words. We are also trying to apply similar ideas to predictive modelling based on retrospective clinical data, in particular using unsupervised topic modelling methods on narrative patient records for outcome prediction and risk stratification for different patient populations.’
PROFILE
Anna Rumshisky PhD studied Computer Science at Brandeis University until 2009. As an Assistant Professor at the Department of Computer Science at University of Massachusetts, Lowell and research affiliate at the Clinical Decision Making group at the Computer Science and Artificial Intelligence Laboratory at MIT, her primary research area is natural language processing (NLP), with applications in clinical informatics, computational lexical semantics, as well as digital humanities and social science. Her work focuses on the development of data-informed unsupervised learning methods and on leveraging existing resources and information-harvesting techniques to overcome the knowledge acquisition bottleneck.
04.03.2013