Image source: Pete Linforth from Pixabay
News • Pandemic forecast modelling
Exploring the uncertainties in Covid-19 simulations
Computer modelling to forecast Covid-19 mortality contains significant uncertainty in its predictions, according to an international study led by researchers at University College London (UCL) and Centrum Wiskunde & Informatica (CWI) in the Netherlands.
While in a physical experiment it is common practice to provide error bars along with the measured values, the predictions from a computer model often lack a measure of uncertainty. This is despite the fact that such models undeniably are uncertain, and are used in high-level decision making. The international research team argues that computational predictions without error bars can paint a very incomplete picture, which they demonstrated in a recent study with a computer model used for evaluating Covid-19 intervention scenarios.
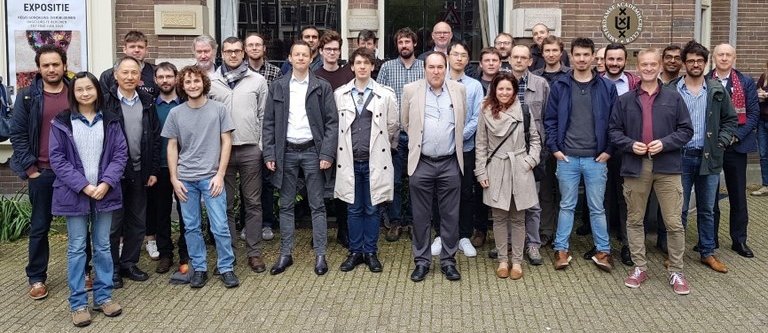
Picture: VECMA
This study was done within the VECMA project, a European Union Horizon 2020 research and innovation programme. At CWI, researchers Wouter Edeling and Daan Crommelin from the Scientific Computing group were involved. Edeling, first author of the article, and a specialist in quantifying modelling uncertainties, made part of the software for the VECMA toolkit. This was used to couple a uncertainty quantification (UQ) technique to the well-known epidemiological ‘CovidSim’ model from Neil Ferguson of Imperial College in the UK and data from the UK from 2020.
Having many parameters means that the computational costs will be inordinately high – often referred to as the ‘curse of dimensionality’
Wouter Edeling
Edeling explained: “For models with a high number of parameters like CovidSim, it is very difficult to study which effect uncertainties in the input parameters have on uncertainties in the output. Having many parameters means that the computational costs will be inordinately high – often referred to as the ‘curse of dimensionality’. We investigate how to do the computations as efficiently as possible, by finding out which parameters matter most for the output uncertainties. By focusing on these parameters it becomes possible to make good probabilistic predictions, which can be used by governments for their decisions.”
The new methods are very effective. In testing the robustness of CovidSim the research team found that, although the code contained 940 parameters, 60 were important and, of those, only 19 dominated the variance in the output predictions. Half of the overall variation in their results was down to just three of the 940 input parameters: the disease’s latency period, the delay in an infected person self-isolating, and the effectiveness of social distancing. Whereas the latency period is a biological parameter, the other two (and quite a few others which were influential) are related to the intervention scenarios and human behavior. While they represent a difficult modeling task, unlike the biological aspects, these parameters (and the phenomena which they model), can be influenced by governmental policy.
Daan Crommelin, group leader of CWI’s Scientific Computing group and professor at UvA , says: "It is important to know about the uncertainties of computer simulations and model predictions. Not only for simulations of the pandemic but also for example in environmental studies, weather and climate modeling, or economics. It may be tempting to focus on a single prediction or a single simulation outcome, but it can also be very relevant to see how wide the range of possible outcomes is."
Source: Centrum Wiskunde & Informatica (CWI)
26.02.2021